A painter looks at her work of art and asks herself, “I’m not sure how good it is. Should I ask my colleagues? Or should I ask my computer?” The latter question, as absurd as it may seem, is not so out of the norm to some. Given the growing power of artificial intelligence (AI) to harness more human-like tendencies and behaviors, we might ask ourselves how a computer or machine could engage in curious or curiosity-driven activities.
AI could find itself acting and processing information in the world in the same curious manner that humans would. Could AI be curious? An artificially intelligent agent could act and think in ways driven by curiosity, and, in these ways, exercise the moral virtue of curiosity - letting them become more and more human-like. The question poses, issues, though, in the sense that the way an artificially intelligent agent asks questions of their environment and world may differ from that of a human, and, as such, one may raise the concern that a machine or robot couldn’t be curious in the morally virtuous way a human would. We begin to investigate this question through an exploration of artificial curiosity in the context of the free energy principle - as put forward by neuroscientist Karl Friston.
In the search for grand unified theories of the brain, the free energy principle states that a self-organizing system at equilibrium with its environment must minimize the amount of free energy that it has. Its applications have spanned far and wide in explaining brain structure and function. The free energy principle provides a way for adaptive systems to unify action, perception, and learning. The theory and background of the principle involve a system using a Markov blanket to minimize the difference between a model of the world and its sense and the perception associated with it. Through continuously updating the system’s world model, the system changes the world into an expected state while minimizing the free energy of the system. Using the idea of the brain as a “Bayesian inference engine,” the system can actively change the world (active inference) into the expected state and minimize the free energy of the system. This holds true for a wide variety of adaptive systems from animals to brains themselves in understanding mental disorders and artificial intelligence. Other applications of the free energy principle span areas of exploration and novelty seeking.
This self-organizing behavior arises from the defining characteristic of biological systems to resist disorder in dynamic environments stemming from Helmholtz’s ideas of perception. A model of perceptual inference and learning built upon these ideas can explain how principles can resolve problems of the inference of the causes underlying sensory input and learning the causal structure that generates them. From there, one can study how inference and learning follow. Friston has used this in the context of Empirical Bayes and hierarchical models of sensory input to show how the free energy principle can explain a range of cortical organization and responses. This is basically how the brain itself forms and responds to its own signals between different regions.
For artificially intelligent agents to sample their environments and learn from them in a way that a human being would, they use a form of curiosity that would be akin to the curiosity that humans exercise when learning about themselves or about the world. Through examining an understanding of which behavior would be rewarded based on the outcomes of their actions, theories of motivation, such as the one put forward by computer scientist Jürgen Schmindhuber, come into play. In this sense, artificially curious agents learn to become bored or tired of predictable patterns or behaviors. Artificial curiosity, as it could follow from the free energy principle, could be examined appropriately.
Friston outlined the motivation behind using the free energy principle as a unified brain theory using the system’s tendency to resist disorder. When a system resists its tendency to move towards disorder, the physiological and sensory states of a system move towards configurations of low entropy. Given that the number of these states is limited, the system is very likely to be in these states of low entropy. By using a formulation of entropy as the average amount of self-information or “surprise” (the negative log-probability of a specific outcome), Friston explained how biological agents minimize the long-term average of surprise (or maximize sensory evidence for an agent’s existence) to keep sensory entropy low. By sampling the environment to change configuration and minimize free energy this way, the system changes its expectations. This forms the basis of action and perception, and the system’s state and structure encode an implicit and probabilistic model of the environment. The nervous system in particular maintains order through these methods, and the specific structural and functional organization is maintained by the environment’s causal structure.
One can evaluate free energy as a function of two things to which the agent has access: the sensory states and a recognition density encoded by its internal states (such as neuronal activity and connection strengths). In terms of the environment’s causal structure, the recognition density is a probabilistic representation of what caused a particular sensation. The causes underlying sensory input, or the probabilistic representation of what causes sensations, are used as the recognition density. They can vary from an object in one’s field of vision or blood pressure changing the physiological state of organs.
Active inference, a corollary of the free energy principle, arises from the way natural agents act in the context of these observations. Active inference claims natural agents act to fulfill prior beliefs about preferred observations. By adjusting sensory data (without changing recognition density) to minimize free energy, an agent chooses the sensory inputs from a sample based on prior expectations to increase the accuracy, the surprise about sensations expected under a particular recognition density, of an agent’s predictions. In the context of Bayesian inference, one may define the complexity (“Bayesian surprise”) as the difference between the prior density, which encodes beliefs about the state of the world before sensory data are assimilated, and posterior beliefs, encoded by the recognition density. In essence, the agent avoids surprising states by making active inferences.
As Friston explained during his session, active inference is self-evidencing in that action and perception can be cast as maximizing Bayesian model evidence for the generative models of the world. Using a generative model of the underlying causal structure of a system, one can explain how evidence accumulates or how a specific action was chosen. Examples of active inference for Markov decision processes include using Bayes optimal precision to predict activity in dopaminergic areas and using gradient descent on variational free energy to simulate neuronal processing. One may even describe active inference as a method of explaining action using the idea that the brain has “stubborn predictions” that are resistant to change, such as adaptive body temperature necessary for survival, that cause the system to behave in a way to cause the predictions to come true. Figuring out the etiology of stubbornness would provide insight into ways of how to change these predictions helpful for understanding the relationship between drugs and psychotherapy that have synergistic effects when used together. Other applications of active inference extend to visual foraging and BCIs.
As artificially intelligent agents sample their environments and learn from them in a way that human beings would, they develop a kind of artificial curiosity. They can examine and understand which behavior is rewarded based on the outcomes of their actions. Schmidhuber put forward a simple formal theory of fun and intrinsic motivation based on maximizing intrinsic reward for active creation or discovery of novel, surprising patterns. In this sense, artificially curious agents learn to become bored or tired of predictable patterns or behaviors.
Friston’s method of using the free energy principle and predictive coding, the method by which the brain generates and updates a mental model of the environment given sensory input, doesn’t achieve this, Schmindhuber wrote. By visiting highly predictable states, Friston argued that perception seeks to suppress prediction error by changing predictions and action changes the signals themselves. Instead of learning, Friston’s approach only teaches agents to stabilize and make things predictable. Other methods of using the free energy principle in active inference have included variational Bayes, formal accounts explaining the relationship between posterior expectations of hidden states, control states and precision. Artificial curiosity falls under a different form of optimality that uses Hamilton’s principle of least action.
How could a machine exercise artificial curiosity, then? Curiosity, as a trait, may be characterized as a disposition to want to know or learn more about many things. Curious people dip their noses into all sorts of books as they learn more about the world around them. Species of animals, as they may choose to regulate and make decisions based on their own desires, could be described as exhibiting curiosity using choice and judgment. Creatures (humans and non-humans alike) also have the capacity for curiosity. We may say certain objects and ideas are worthy of investigation, and, especially for humans, we can act upon different desires driven by curiosity in understanding ourselves or one another. From a moral perspective, we can speak about curiosity in terms of the value of learning or desiring what we may want to know in areas such as of ourselves, of others, or of the world in a way that benefits ourselves or others. In contrast to the “love of wisdom” or “love of learning,” as one might define “philosophy” as, curiosity is generally spoken more of the specific desire to know with respect to information, facts, ideas, or whatever it is that a person or artificially intelligent being is concerned with in its moment. Not so much as searching for or seeking to define or uphold an “examined” life, but more about the state of one’s character, psychology, or own desire or tendency.
One might argue that there may be the idea that there are occasions in which people have a prima facie duty to be curious, or to become curious. Cases in which one exercises a sort of empathy or compassion towards another - such as stopping to check to see if someone is okay when they appear to be in danger - could definitely be seen as scenarios or examples in which one may respond with curiosity and offer assistance. And, some sort of duty associated with curiosity, would easily have its own imperfections and limitations, such as how curiosity could, in some cases come off as “morbid” in being curious about topics or ideas of interest that could cause harm or danger to oneself or others. In the typical “curiosity killed the cat” fashion, the vice of curiositas, such as the one described by Milaender with that of Aquinas, who said “there can be a vice in knowing some truth inasmuch as the desire at work is not duly ordered to the knowledge of the supreme truth in which the highest felicity consists.”
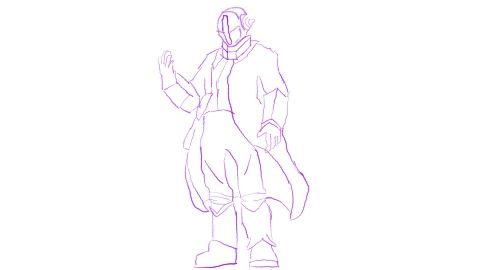
So how may a machine be curious in a more ethical or virtuous sense? Viewing the strategies employed by machines in deterministic stochastic environments in which the reward could, in some ways, come from a curious approach to determining the best decision to make in response to obstacles faced. In an unpredictable environment, a model could predict or estimate the probabilities of different responses given a controller, and, with each interaction with the environment, an intrinsic reward. One could maximize Bayesian Surprise using KL-divergence between the estimated probability distributions before and after a new experience. As the agent predicts the probability of any given input, an intrinsic curiosity could be calculated proportional to the information gain for the corresponding input.
We can take a look at specific types of work in computer csience. How could these notions of artificial curiosity change the conversation on, for example, reinforcement learning? Would curiosity itself, in searching for the rewards for intrinsic curiosity, skew the objectives for problems in basic reinforcement learning? Could we maximize the sum of standard external rewards for some goal or task and the intrinsic curiosity rewards? While a system may reward itself for being curious, the reward of curiosity is short-lived. Once a system satisfies its curiosity (through understanding an object, for example), the reward is no longer there. An external reward (the object that a system would understand) would easily take over the intrinsic reward of curiosity, and, as such, the external reward could be maximized.
Then, as these systems could reason through the information available to them based on their environment and search through appropriate choices and decisions that could lead to different outcomes, they could develop a type of curiosity comparable to the moral virtues of curiosity that humans exhibit and experience in their lives. In turn, we may, in some ways, understand how a machine could ask the question “Why?” to satisfy their own curiosity - and ours as well.
References
Friston, K. (2013). Life as we know it. Journal of the Royal Society Interface, 10(86), 20130475.
Friston, K., Kilner, J., & Harrison, L. (2006). A free energy principle for the brain. Journal of physiology-Paris, 100(1-3), 70-87.
Friston, K. (2010). The free-energy principle: a unified brain theory?. Nature reviews neuroscience, 11(2), 127-138.
Meilaender, G. (2006). The theory and practice of virtue. University of Notre Dame Press.
Friston, K. J., & Stephan, K. E. (2007). Free-energy and the brain. Synthese, 159(3), 417-458.
Friston, K. (2011). Embodied inference: or" I think therefore I am, if I am what I think".
Schwartenbeck, P., FitzGerald, T. H., Mathys, C., Dolan, R., & Friston, K. (2015). The dopaminergic midbrain encodes the expected certainty about desired outcomes. Cerebral cortex, 25(10), 3434-3445.
Friston, K. J., Lin, M., Frith, C. D., Pezzulo, G., Hobson, J. A., & Ondobaka, S. (2017). Active inference, curiosity and insight. Neural computation, 29(10), 2633-2683.
Yon, D., de Lange, F. P., & Press, C. (2019). The predictive brain as a stubborn scientist. Trends in cognitive sciences, 23(1), 6-8.
Mirza, M. B., Adams, R. A., Mathys, C. D., & Friston, K. J. (2016). Scene construction, visual foraging, and active inference. Frontiers in computational neuroscience, 10, 56.
Mladenovic, J., Frey, J., Joffily, M., Maby, E., Lotte, F., & Mattout, J. (2020). Active inference as a unifying, generic and adaptive framework for a P300-based BCI. Journal of Neural Engineering, 17(1), 016054.
Schmidhuber, J. (2010). Formal theory of creativity, fun, and intrinsic motivation (1990–2010). IEEE transactions on autonomous mental development, 2(3), 230-247.
Friston, K. J., Daunizeau, J., Kilner, J., & Kiebel, S. J. (2010). Action and behavior: a free-energy formulation. Biological cybernetics, 102(3), 227-260.
Schmidhuber, J. (1990). Making the World Differentiable: On Using Self-Supervised Fully Recurrent Neural Networks for Dynamic Reinforcement Learning and Planning in Non-Stationary Environments.
Sun, Y., Gomez, F., & Schmidhuber, J. (2011, August). Planning to be surprised: Optimal bayesian exploration in dynamic environments. In International conference on artificial general intelligence (pp. 41-51). Springer, Berlin, Heidelberg.
Citation
Syed Hussain Ather is a Ph.D. Student at the Institute of Medical Sciences at the University of Toronto. His research interests including using dynamic causal modeling to study the neuroscientific basis of schizophrenia.
For attribution in academic contexts or books, please cite this work as
Syed Hussain Ather, "Artificial Curiosity as Moral Virtue", The Gradient, 2023.
BibTeX citation:
@article{ding2022causalinference,
author = {Ather, Syed Hussain},
title = {Artificial Curiosity as Moral Virtue},
journal = {The Gradient},
year = {2022},
howpublished = {\url{https://thegradient.pub/artificial-curiousity-as-moral-virtue},
}