Since the discovery of DNA in the 1950s, biologists have sought to tie lengths of genetic code to a range of cellular parts and processes—including, for example, the mRNA transcription of specific antibodies that powers the now-famous mRNA vaccines. Despite the progress in sequencing and understanding the genome since the discovery of DNA, one big missing link remained. Biologists lacked a way to accurately and efficiently predict the 3-D shape of an unknown protein using just its DNA or RNA source code. In biology, structure determines function. What a protein does in a cell depends on its shape. Cylindrical with a hollow middle makes for a good membrane receptor, while U-shaped enzymes catalyze chemical reactions in their fjord-like cavities. Being able to predict or even design proteins would be a leap forward in our understanding of human disease and unlock new treatments for a range of diseases.
But for more than 70 years, scientists have been stuck with slow methods that strained computers and relied largely on their own guesswork to tease out a protein’s structure. Despite knowing which stretches of DNA code for each of the amino acids that form the building blocks of every protein, biologists lacked a repeatable, generalizable formula to solve this so-called “protein-folding problem.” They needed a systematic understanding of how any string of amino acids, once linked, would fold into a 3-dimensional shape to unlock the vast universe of proteins.
In 2020, Google’s AI team DeepMind announced that its algorithm, AlphaFold, had solved the protein-folding problem. At first, this stunning breakthrough was met with excitement from most, with scientists always ready to test a new tool, and amusement by some. After all, wasn’t this the same company whose algorithm AlphaGo had defeated the world champion in the Chinese strategy game Go, just a few years before? Mastering a game more complex than chess, difficult as that is, felt trivial compared to the protein-folding problem. But AlphaFold proved its scientific mettle by sweeping an annual competition in which teams of biologists guess the structure of proteins based only on their genetic code. The algorithm far outpaced its human rivals, posting scores that predicted the final shape within an angstrom, the width of a single atom. Soon after, AlphaFold passed its first real-world test by correctly predicting the shape of the SARS-CoV-2 ‘spike’ protein, the virus’ conspicuous membrane receptor that is targeted by vaccines.
The success of AlphaFold soon became impossible to ignore, and scientists began trying out the algorithm in their labs. By 2021 Science magazine crowned an open-source version of AlphaFold the “Method of the Year.” Biochemist and Editor-in-Chief H. Holden Thorp of the journal Science wrote in an editorial, “The breakthrough in protein-folding is one of the greatest ever in terms of both the scientific achievement and the enabling of future research.” Today, AlphaFold’s predictions are so accurate that the protein-folding problem is considered solved after more than 70 years of searching. And while the protein-folding problem may be the highest profile achievement of AI in science to date, artificial intelligence is quietly making discoveries in a number of scientific fields.
By turbocharging the discovery process and providing scientists with new investigative tools, AI is also transforming how science is done. The technology upgrades research mainstays like microscopes and genome sequencers , adding new technical capacities to the instruments and making them more powerful. AI-powered drug design and gravity wave detectors offer scientists new tools to probe and control the natural world. Off the lab bench, AI can also deploy advanced simulation capabilities and reasoning systems to develop real-world models and test hypotheses using them. With manifold impacts stretching the length of the scientific method, AI is ushering in a scientific revolution through groundbreaking discoveries, novel techniques and augmented tools, and automated methods that advance the speed and accuracy of the scientific process.
Beyond the protein-folding problem, AI has proven its scientific worth with discoveries in a number of fields, from cosmology and chemistry to semiconductor design and materials science. For example, the team at DeepMind developed another algorithm that calculated the electron density of molecules, beating the shortcut method scientists have relied on for 60 years. Knowing the electron density of a given molecule goes a long way towards understanding the material’s physical and chemical properties. But because electrons are governed by quantum mechanics, calculating the density of a specific electron requires complex equations and quickly turns into a computational nightmare. Instead, scientists used the average density of a material's electrons to guide them, sidestepping the difficult quantum calculations. However, DeepMind’s algorithm tackles the quantum side directly and has proven to be more accurate than the shortcut method. Similar to the protein-folding problem, AI was able to surpass the method scientists had used for decades and unlock new methods for accurately predicting physical and chemical properties.
AI’s impact on science extends beyond the new finds by the technology in four key ways. First, AI can quickly read through the scientific literature, allowing it to learn the fundamental rules, facts, and equations of science, and help scientists manage the flood of papers and data that is drowning every field. In 2020 alone, between 100,000 and 180,000 articles were published in scientific journals about COVID-19. And while it makes sense that researchers would focus on something as urgent as an ongoing global pandemic, papers about COVID-19 only made up about 4-6% of the total articles on the largest biomedical database. The wave of papers and data being produced far exceeds any scientists’ ability to read them, leaving researchers unable to truly keep pace with innovations in their fields.
That is where AI comes in. In drug chemistry, for example, the company Insilico is entering Phase I clinical trials for its wholly-AI-designed drug designed to tackle a disease known as Idiopathic Pulmonary Fibrosis (IPF). Insilico’s algorithm picks disease targets by reading through the medical literature to find potential proteins, cells, or pathogens to pinpoint. Once a target is selected, the algorithm can design a therapeutic to treat the disease. Insilico has developed an end-to-end AI platform for drug discovery that can automatically stay on top of the latest results and data in the field so that scientists can be informed without being overwhelmed.
Second, scientists are also faced with a deluge of data as their instruments get more precise and their findings dig deeper into nature. AI can help here, too. A team of scientists at Argonne National Laboratory developed an algorithm that can make sense of gravitational waves–ripples in the fabric of the space-time continuum that was predicted by Einstein but not discovered until 2015. This algorithm processed a month’s worth of data in just seven minutes, providing an accelerated, scalable, and reproducible detection of gravitational waves. Even better, the algorithm can also be run on a standard graphics processing unit (GPU), freeing researchers from needing specialized equipment to collect and interpret gravitational waves data. ‘“What’s exciting to me about this project,”’ said Ian Foster, director of Argonne’s Data Science and Learning (DSL) division, “is that it shows how, with the right tools, AI methods can be integrated naturally into the workflows of scientists — allowing them to do their work faster and better — augmenting, not replacing, human intelligence.”’ With AI, what was once a flood of data is now a controllable stream of information that accelerates the pace of science.
Third, AI has been quietly upgrading some of the longtime mainstays of any lab: microscopes and DNA sequencers. At Argonne National Labs, researchers have found a way to boost what information an electron microscope can retrieve about the sample, while also increasing the resolution and sensitivity of the instrument. Electron microscopes differ from the kind many are familiar with in high school or college biology classes in that they do not rely on visible light to construct images. Instead, as the name suggests, they use electrons, which allows them to take images at higher resolutions and finer detail than other microscopes. Researchers at Argonne National Labs devised a way to record the phase data, which relays key information about a sample’s physical and chemical properties, on an electron microscope using AI, boosting the power and capacity of the instrument.
Somewhat similarly, another upgrade courtesy of AI is found in so-called light-field microscopy, which takes moving images in high-definition 3-D. It typically takes scientists days to reconstruct the movies, but with AI, the time it takes to process such high-resolution data in motion shrinks to just seconds without losing resolution or detail. DNA sequencers, the workhorses of the genomic era, have also been augmented by AI. Earlier this year, a team of scientists used AI to halve the time it takes to sequence DNA and hope to halve it again soon. Put simply, AI is upgrading even the most basic of scientific tools.
Finally, where AI really shines in the lab is simulating complex systems, making it an increasingly standard tool in basic science research. Last year, researchers showed off AI’s multidisciplinary capability by building groundbreaking simulations in ten scientific fields from physics and astronomy to geology and climate science. All ten emulators were trained from the same deep neural network, called DENSE, and sped up simulations by as much as a billion times when compared to other methods—all while remaining just as accurate. Crucially, emulators can be used to solve “inverse problems,” where a researcher know the result but wants to find out what variables would cause the output. AI excels at this kind of calculation and can easily unravel the path to a particular answer.
As useful as simulations are, researchers also want to ensure their models hold up in the real world. Two of the leading tech companies, Google and Samsung, recently turned to AI to plan the layout of some of their chips. Google concluded that the AI-designed chip was, ‘“superior or comparable to those produced by humans in all key metrics, including power consumption, performance, and chip area.”’ Going further, the company used that AI to design its next generation of AI accelerators (TPUs, chips made for AI rather than the standard CPU or GPU). Likewise, Samsung relied on AI chip design software to create Exynos, a chip in their wearable products and cars. With its high-fidelity simulations, AI is delivering scientists a powerful tool that is revolutionizing how they model and experiment with the natural world.
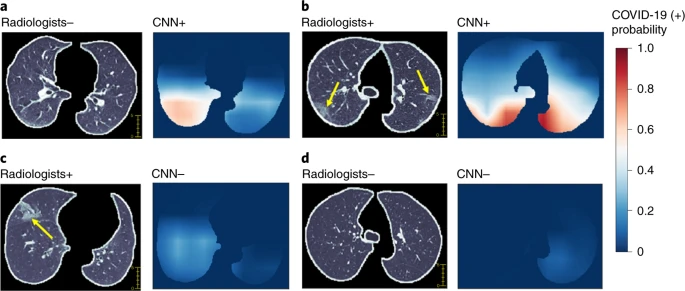
There has been no better real-world test for AI’s modeling capacity than the COVID-19 pandemic. First, AlphaFold, the protein-folding algorithm, correctly predicted the prominent ‘spike’ protein, showing how AI could accelerate the development of vaccines or therapeutics in a future pandemic. But perhaps even more impressive, in the summer of 2020, Japanese scientists used the world’s most powerful supercomputer, Fugaku, to model the spread of COVID-19 in the air. Backed by deep neural networks and thousands of GPUs, Fugaku provided the world with decisive evidence that the virus was air-borne and convinced the WHO to change its guidance for controlling COVID-19 accordingly (e.g., masking, ventilation, and the risk of indoor vs outdoor activities). In the real world, AI has proved its worth by informing global mitigation strategies during a crisis.
In addition to making new discoveries and adding new tools to the scientific arsenals, AI also find patterns in data, makes testable predictions, and uses them to incorporate new evidence into its model, mirroring the scientific method. The philosopher Karl Popper popularized the idea that science advanced by discarding falsifiable hypotheses–predictions that could be experimentally tested and proven false–and that this process of elimination through theory and experiment was a hallmark of the scientific method. As some of AI’s recent breakthroughs show, the technology also generates hypotheses that can be experimentally tested and, by process of elimination, provide a rigorous and falsifiable answer. DeepMind’s electron density model beat researchers by approximating the scientific process and iterating feedback between prediction and experiment until it had a better grasp of the quantum calculations. AI solved the protein folding problem by testing its model on thousands of experimentally determined proteins, refining its guesses and pruning its neural network by giving more weight to branches that are closer to the solution. And after researchers built an open-source version of AlphaFold, other scientists have used the model to unlock how RNA structures fold and how proteins bind together.
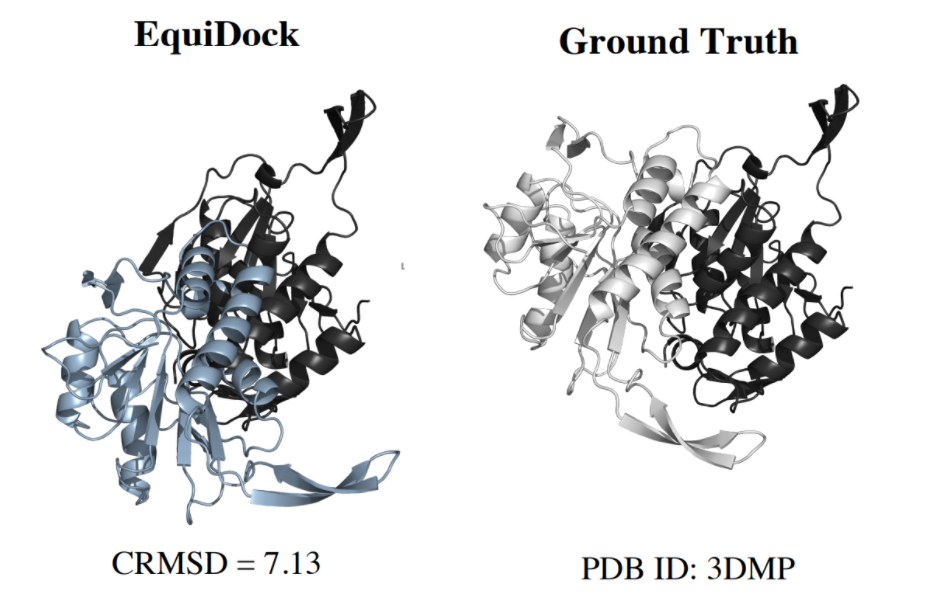
Overall, understanding protein binding opens the door to powerful new drugs because many reactions in the cell are the result of proteins working together. Both advances unlock a new era of therapeutic design that utilizes an end-to-end AI pipeline to target diseases and design precision treatments for them. For example, a research team from Huawei’s AI lab used a version of the model to automatically generate antibodies against a target infection. From drug design to protein binding, AI’s ability to model, analyze, and control nature will only continue to grow.
DeepMind’s most recent breakthrough applied AI to the problem of containing and sustaining a nuclear fusion reaction. The AI successfully controlled the same reaction of melding hydrogen and helium that powers every star in the universe for a record length of time and discovered new shapes in which to stably hold the plasma. The experiment was a major step towards developing viable fusion power, which could provide enough renewable energy to power the world. This case highlights the most promising use of AI in science: it sees patterns that we cannot and analyzes our environment from a divergent but complementary perspective. Working together with researchers and anchored in the scientific method, AI is capable of the same kind of exploratory problem-solving that draws on iterative theory and experiment that undergirds the scientific process.
Science may be best described as an exploration of the unknown. And on this journey, AI is a partner that perceives the natural world and its unexplored parts differently than we do, opening new ways of understanding and harnessing the power of our world. As Hiroaki Kitano, Director of Research at Sony, describes it, scientific discovery is a “search” problem, based on a self-correcting system of hypotheses, experiments, and data that AI can emulate. Through automation and algorithms, AI can link together causes and correlations that no human could connect. But AI is more than a just powerful tool in the hands of scientists and partner on this search. The technology is also transforming the scientific process, automating and adding to what people can accomplish using it. AI is ushering in a new scientific revolution by making remarkable breakthroughs in a number of fields, unlocking new approaches to science, and accelerating the pace of science and innovation. As partners in discovery, AI and scientists can explore more of science’s endless frontier together than either could alone.
Author Bio
Bryan McMahon is an AI Policy Researcher at NEDO, the Japanese government’s science and technology R&D organization, where he analyzes US and international AI policy strategies. He earned a B.A. in Biology from Duke University, where he studied science policy in its Science and Society Program, a research institute sitting at the intersections of science, law, and policy. Previously, his work on artificial intelligence policy has been published in the Journal of Science Policy and Governance as well as a recent piece analyzing the rules governing so-called ‘killer robots’ in Skynet Today.
Citation
For attribution in academic contexts or books, please cite this work as
Bryan McMahon, "AI is Ushering In a New Scientific Revolution", The Gradient, 2022.
BibTeX citation:
@article{mcmahon2022revolution,
author = {McMahon, Bryan},
title = {AI is Ushering In a New Scientific Revolution},
journal = {The Gradient},
year = {2022},
howpublished = {\url{https://thegradient.pub/ai-scientific-revolution} },
}