Maybe every paper abstract should have a mandatory field of what the limitations of the proposed approach are. That way some of the science miscommunications and hypes could maybe be avoided.
— Sebastian Risi (@risi1979) October 28, 2019
The media is often tempted to report each tiny new advance in a field, be it AI or nanotechnology, as a great triumph that will soon fundamentally alter our world. Occasionally, of course, new discoveries are underreported. The transistor did not make huge waves when it was first introduced, and few people initially appreciated the full potential of the Internet. But for every transistor and Internet, there are thousands or tens of thousands of minor results that are overrreported, products and ideas that never materialized, purported advances like cold fusion that have not been replicated, and experiments that lie on blind alleys that don't ultimately reshape the world, contrary to their enthusiastic initial billing.
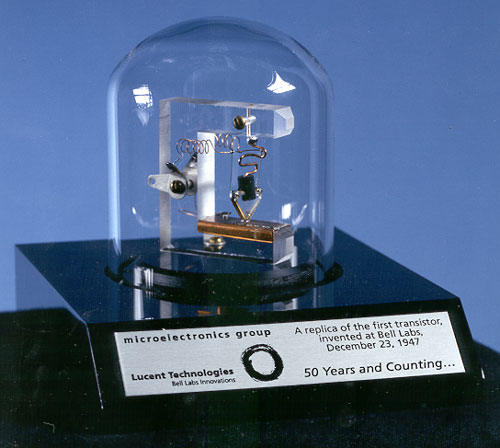
Part of this of course, is because the public loves stories of revolution, and yawns at reports of minor incremental advance. But researchers are often complicit, because they too thrive on publicity which can materially impact their funding and even their salaries. For the most part, both media and significant fraction of researchers are satisfied with a status quo in which there is a steady stream of results that are first over-hyped, then quietly forgotten.
Consider three separate results from the last several weeks that were reported in leading media outlets in ways that were fundamentally misleading:
-
Earlier this week on November 24th, The Economist published an interview with OpenAI's GPT-2 sentence generation system, misleadingly said that GPT-2’s answers were “unedited”, when in reality each answer that was published was selected from five options, filters for coherence and humor. This led the public to think that conversational AI was much closer than it actually is. This impression may have been inadvertently [see update below] furthered when leading AI expert (Erik Bryjngjolffson) tweeted that the interview was “impressive” and that “the answers are more coherent than those of many humans.” In fact the apparent coherence of the interview stemmed from (a) the enormous corpus of human writing that the system drew from and (b) the filtering for coherence that was done by the human journalist. Brynjjolffson issued a correction, but, in keeping with the theme of this piece, retweets of his original tweet outnumbered tweets of his correction by about 75:1 - evidence that triumphant but misleading news travels faster than more sober news.
-
OpenAI created a pair of neural networks that allowed a robot to learn to manipulate a custom-built Rubik's cube, and publicized it with a somewhat misleading video and blog that led many to think that the system had learned the cognitive aspects of cube-solving (viz. which cube faces to turn when) when it had not in fact learned that aspect of the cube-solving process. (Rather, cube-solving, as apart from dexterity, was computed via with a classical, symbol-manipulating cube-solving algorithm devised in 1992 that was innate, not learned). Also less than obvious from the widely circulated video was the fact that the cube was instrumented with Bluetooth sensors, and the fact that even in the best case only 20% of fully-scrambled cubes were solved. Media coverage tended to miss many of these nuances. The Washington Post for example reported that “OpenAI’s researchers say they didn’t “explicitly program” the machine to solve the puzzle”, which was at best unclear. The Post later issued a correction -- “Correction: OpenAI focused their research on physical manipulation of a Rubik’s Cube using a robotic hand, not on solving the puzzle…” -- but one again suspects that the number of people who read the correction was small relative to those who read and were misled by the original story.
- At least two recent papers on the use of neural networks in physics were widely overreported, even by prestigious outlets such as Technology Review. In both cases, neural networks that solved toy versions of complex problem were lauded in a fashion disproportionate to the actual accomplishment. For example, one report claimed that “A neural net solves the three-body problem 100 million times faster” than conventional approaches, but the network did no solving in the classical sense, it did approximation, and it approximated only a highly simplified two degree-of-freedom problem (rather than the conventional 10), and only with objects of identical mass. The original tech review story was widely distributed around the web; a subsequent, detailed critique by Ernest Davis and myself in Nautilus received significant attention, but glancing at retweets as a crude metric I still would not be surprised if the ratio of those reading the original breathless report relative to those reading our more sober analysis was again on the order of 75:1, if not even more skewed.
Unfortunately, the problem of overhyped AI extends beyond the media itself. In fact, for decades, since AI’s inception many (though certainly not all) of the leading figures in AI have fanned the flames of hype.
This goes back to early founders who believed that we might now call artificial general intelligence (AGI) was no more than a couple decades away. In 1966, the MIT AI lab famously assigned Gerald Sussman the problem of solving vision in a summer; as we all know, machine vision still hasn't been solved over five decades later. General AI still seems like it might be a couple decades away, sixty years after the first optimistic projections were issued.
This trend continues in the modern era. Here are some examples from the more recent history of AI, from some of its best known contemporary figures
-
In an interview with The Guardian in 2015 entitled “Google a step closer to developing machines with human-like intelligence”, Geoff Hinton, widely regarded as the "Godfather of deep learning", enthused that Google (a company he recently joined) with the new approach would (in The Guardian’s paraphrase) “help crack two of the central challenges in artificial intelligence: mastering natural, conversational language, and the ability to make leaps of logic” and that the company (again The Guardian’s paraphrase), “is on the brink of developing algorithms with the capacity for logic, natural conversation and even flirtation.” Four years later, we are still a long way from machines that can hold natural conversations absent human intervention, to ensure coherence, and no extant system can reason about the physical world in a reliable way.
-
Roughly a year later Hinton claimed that radiologists are like “the coyote already over the edge of the cliff who hasn’t yet looked down”, suggesting “if you work as a radiologist you are like Wile E. Coyote in the cartoon, you're already over the edge of the cliff” and adding that “We should stop training radiologists now. It’s just completely obvious that within five years, deep learning is going to do better than radiologists.” Hinton further echoed this claim in a 2017 interview with the New Yorker. Hundreds of deep learning for radiology companies have been spawned in the meantime, but thus far no actual radiologists have been replaced, and the best guess is that deep learning can augment radiologists, but not, in the near-term replace them. Hinton’s words frightened many radiology departments. The consequences may have been negative; currently in many parts of the world there is a shortage of radiologists.
-
In November 2016, in the pages of Harvard Business Review, Andrew Ng, another well-known figure in deep learning, wrote that “If a typical person can do a mental task with less than one second of thought, we can probably automate it using AI either now or in the near future.” A more realistic appraisal is that whether or not something can be automated depends very much on the nature of the problem, and the data that can be gathered, and the relation between the two. For closed-end problems like board games, in which a massive amount of data can be gathered through simulation, Ng’s claim has proven prophetic; in open-ended problems, like conversational understanding, which cannot be fully simulated, Ng’s claim thus far has proven incorrect. Business leaders and policy-makers would be well-served to understand the difference between those problems that are amenable to current techniques and those that are not; Ng’s words obscured this. (Rebooting AI gives some discussion.)
Meanwhile, the response of investigators whose work has been misrepresented is often silence, or even quiet approval. Open AI’s Chief Scientist llya Sutskever tweeted that "The economists interviews GPT-2 and the interview makes sense". When I asked him whether he stood by his comments after it became clear that the examples in the Economist interview had been cherrypicked, he didn't respond.
A bit over a month earlier OpenAI's CTO, Greg Brockman, did the cherry-picking himself, tweeting that “A GPT-2 written essay was submitted to the Economist's youth essay contest… One judge, who did not know the essay was written by an AI, gave this review: "It is strongly worded and backs up claims with evidence, but the idea is not incredibly original." What he did not note was that some of the the other judges were considerably more negative about the same essay writing for example that the essay “Doesn’t get to the point quick enough; point isn’t novel, too vague, excessive, high number of rhetorical questions” (judge 2) and most damningly (judge 6) that the “the essay does not fundamentally answer the question nor present a single novel idea, is not strongly argued and is not particularly well written/structured. In addition, I do not think it shows a strong understanding of existing climate policy nor of the scientific literature coming out of the IPCC.” Nobody reading Brockman’s tweet (unless they followed through the link and read the full story) would realize that this level of negativity was expressed. (And many in the community were are still rolling their eyes at OpenAI’s original claims that GPT-2 was “too dangerous” to release.)
Other habits on the part of the research community further an inaccurate AI-is-nearly-here narrative. DeepMind for example often writes papers that enthuse about the potential of the work but lack the sort of sections on potential limits that are a staple of the conclusion sections of most serious scientific work. Instead, they often argue by invited inference, suggesting that they are working on hard problems and grand challenges, implying that the techniques that they are using ought to solve other grand challenges as well - without reckoning with the fact that other problems, such as natural language understanding, differ greatly in character from the games that they have been focusing on. Their Nature papers on AlphaGo[1] and StarCraft[2] all follow this strategy, with essentially no discussion of potential limits.
Mercifully, not everyone in the field overrepresents their work; in the last year or so I have seen terrific, balanced talks by Pieter Abbeel and Yoshua Bengio, both noting what deep learning (and deep reinforcement learning) do well, and yet at the same time articulating the challenges ahead, and bluntly acknowledging how far we need to go. (Abbeel emphasized the gap between laboratory work and robots that could work in the real world, Bengio emphasized the need for incorporating causality). I just wish that were the norm rather than the exception. When it’s not, policy-makers and the general public can easily find themselves confused; because the bias tends to be towards overreporting rather than underreporting results, the public starts fearing a kind of AI (replacing many jobs) that does not and will not exist in the foreseeable future.
The Risk of Overpromising
Why should practitioners care? After all, hype for AI grows the pot for everyone, doesn’t it? Public enthusiasm means more dollars into research, and more people working on AI; we will get to artificial general intelligence faster if there are more dollars and more people. What’s the harm?
I see this as a version of the tragedy of the commons, in which (for example) many people overfish a particular set of waters, yielding more fish for themselves in the short term, until the entire population of fish crashes, and everybody suffers. In AI the risk is this: if and when the public, governments, and investment community recognize that they have been sold an unrealistic picture of AI’s strengths and weaknesses that doesn't match reality, a new AI winter may commence. (The first came in 1974 after an earlier cycle of hype and disappointment.)
We have already seen multiple events that in hindsight could turn out to be augurs:
- Chatbots: Facebook promised a system called M in 2015 that was supposed to revolutionize the boundaries of what personal assistants could do. The AI to build what they wanted did not exist at the time, but the project was conceived of as a data play; humans would answer the first batch of questions, and then deep learning would handle the rest. By 2018 the project was canceled. More generally, enthusiasm for chatbots was high in 2015; now it is widely recognized that current AI can only handle limited, bounded conversations, and even then without full reliability. Promises were made, but not delivered upon.
:format(webp):no_upscale()/cdn.vox-cdn.com/uploads/chorus_asset/file/4003002/facebookm.0.jpg)
- Medical diagnosis: IBM Watson wildly overpromised, and ultimately medical partners like the MD Andersen Cancer Institute pulled out because of disappointing results; the project of adapting Watson to medical diagnosis is now seen widely seen as an over-promise. A lot of people were probably initially expecting DeepMind to step into the medical diagnosis breach, given their extraordinary data access and massive computational and intellectual resources. But the reality is that nothing compelling has yet emerged (and DeepMind’s medical portfolio has since shifted over to Google). Even in the simpler case of radiology, which is largely about perception rather than inference, with smaller demands on natural language understanding, putting lab demos into practice has proven difficult.

-
Fake news detectors: In April 2018 Mark Zuckerberg told Congress that AI would come to the rescue in five to ten years, but by May of this year CTO Mike Schroepfer backed down from promising significant progress in the near term. (Davis and I discuss some of the technical challenges here.)
-
Driverless cars: Many expected this by 2020 (and Elon Musk has promised) but the general consensus of the field is that fully autonomous driving is harder than most people expected, still many years off, except in limited conditions (such as ideal weather, minimal pedestrian traffic, detailed maps, etc.).
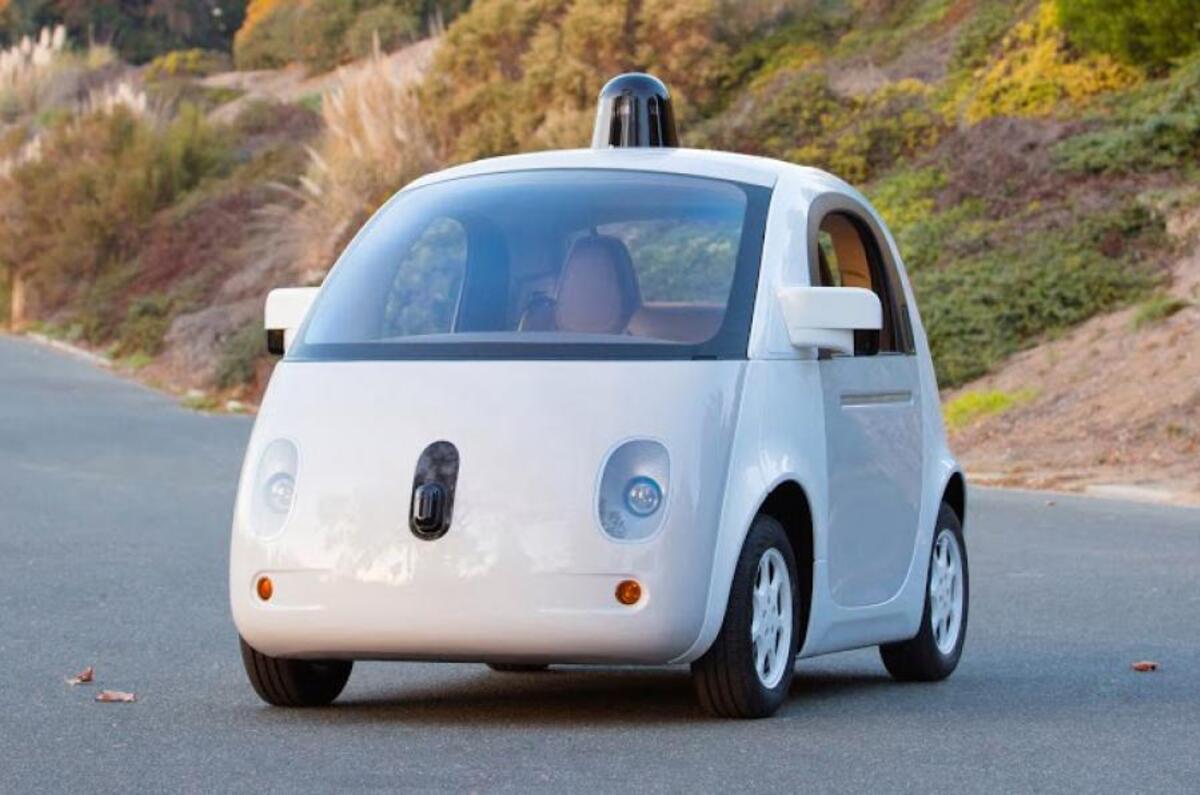
Right now, governments, large corporations and venture capitalists are making massive investments into AI, largely deep learning; if they start to perceive a pattern of overpromising, the whole field might suffer. If driverless cars and conversational bots appear only a year or two late, no problem, but the more deadlines slip, on driverless cars, medical diagnosis and conversational AI, the greater the risk of new AI winter becomes.
Recommendations
To recap thus far, misinformation about AI is common. Although overreporting is not ubiquitous, even prominent media outlets often misrepresent results; corporate interests frequently contribute to the problem. Individual researchers, even some of the most eminent ones, sometimes do as well, while many more sit quietly by, without publicly clarifying, when their results are misinterpreted.
Misinformation is not ubiquitous – some researchers are forthright about limitations, and some new stories are reported accurately, by some venues, with honest recognition of limits, but the overall tendency towards interpreting each incremental advance as revolutionary is widespread, because it fits a happy narrative of human triumph.
The net consequences could, in the end, debilitate the field, paradoxically inducing an AI winter after initially helping stimulate public interest.
In Rebooting AI, Ernie Davis and I made six recommendations, each geared towards how readers - and journalists – and researchers might equally assess each new result that they achieve, asking the same set of questions in a limit section in the discussion of their papers:
- Stripping away the rhetoric, what does the AI system actually do? Does a “reading system” really read?
- How general is the result? (Could a driving system that works in Phoenix work as well in Mumbai? Would a Rubik’s cube system work in opening bottles? How much retraining would be required?)
- Is there a demo where interested readers can probe for themselves?
- If AI system is allegedly better than humans, then which humans, and how much better? (A comparison is low wage workers with little incentive to do well may not truly probe the limits of human ability)
- How far does succeeding at the particular task actually take us toward building genuine AI?
- How robust is the system? Could it work just as well with other data sets, without massive amounts of retraining? AlphaGo works fine on a 19x19 board, but would need to be retrained to play on a rectangular board; the lack of transfer is telling.
A little bit of constructive self-criticism at the end of every research report and media account, not always absent but too frequently missing, might go a long way towards keeping expectations realistic.
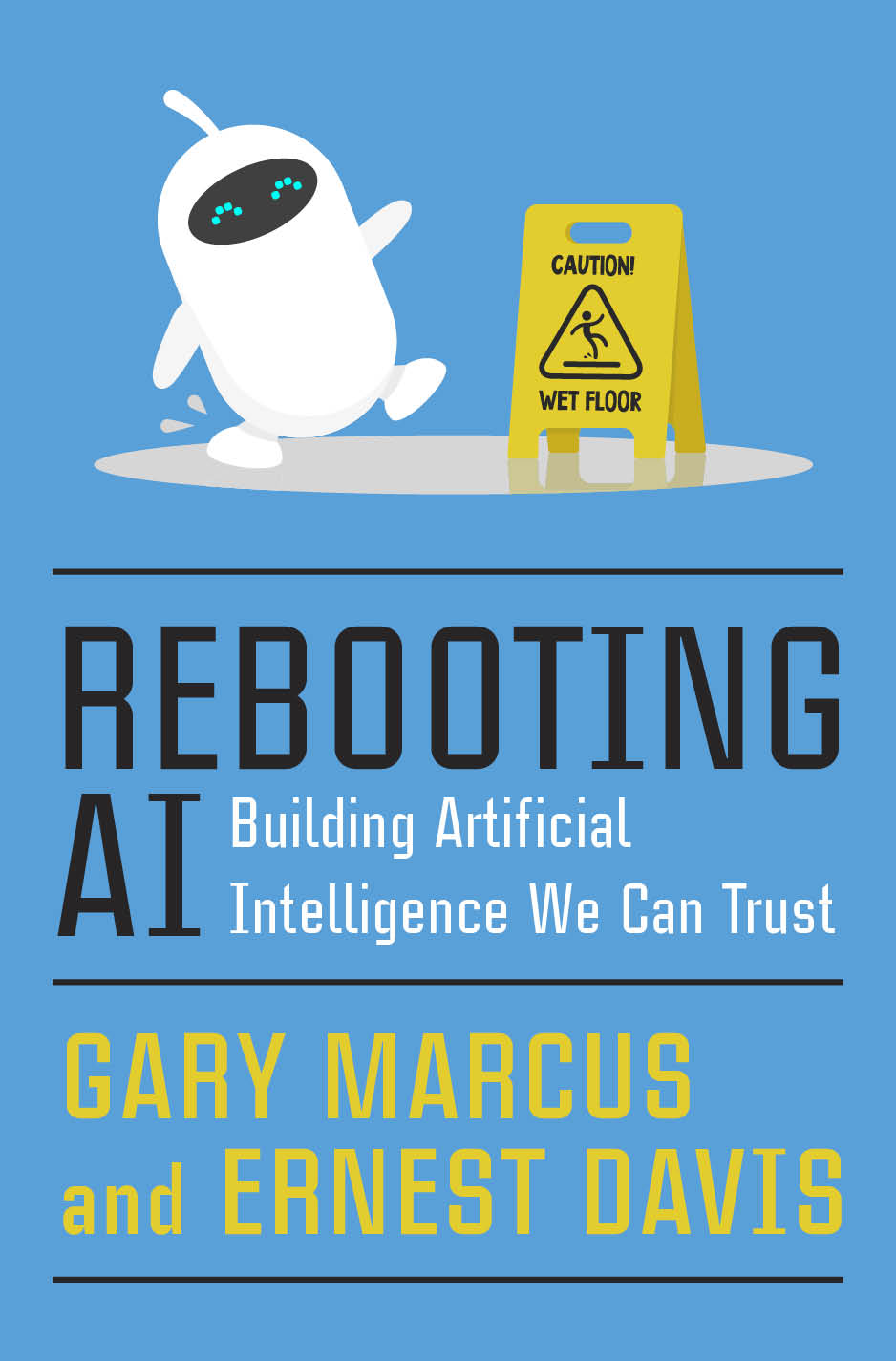
Update: Not long after this piece was published, Erik Brnygjolfsson and I had a spirited discussion of OpenAI's GPT-2 system. He made it very clear that he (like me) doesn't consider GPT-2 to be anything close to artificial general intelligence -- it is capable of coherence in short spurts but not over longer periods of time -- but he also argued persuasively that the GPT-2 system might (despite its limitations in reasoning and longer-term coherence) have important economic or societal implications, eg it might well already be used for troll farms and was recently used effectively for joke writing; I fully agree.
Update 2: Yann LeCun objected to a paragraph referring to a 2015 report in Wired in which he was mentioned, discussing the future of robotics; having investigated the origins of that piece, I think that it is possible that the report's headline misrepresented his position. I have therefore removed the paragraph in question.
David Silver, Aja Huang, Chris J. Maddison, Arthur Guez, Laurent Sifre, George van den Driessche, Julian Schrittwieser, Ioannis Antonoglou, Veda Panneershelvam, Marc Lanctot, Sander Dieleman, Dominik Grewe, John Nham, Nal Kalchbrenner, Ilya Sutskever, Timothy Lillicrap, Madeleine Leach, Koray Kavukcuoglu, Thore Graepel & Demis Hassabis, "Mastering the game of Go with deep neural networks and tree search", Nature volume 529, pp. 484–489 (2016) ↩︎
Oriol Vinyals, Igor Babuschkin, Wojciech M. Czarnecki, Michaël Mathieu, Andrew Dudzik, Junyoung Chung, David H. Choi, Richard Powell, Timo Ewalds, Petko Georgiev, Junhyuk Oh, Dan Horgan, Manuel Kroiss, Ivo Danihelka, Aja Huang, Laurent Sifre, Trevor Cai, John P. Agapiou, Max Jaderberg, Alexander S. Vezhnevets, Rémi Leblond, Tobias Pohlen, Valentin Dalibard, David Budden, Yury Sulsky, James Molloy, Tom L. Paine, Caglar Gulcehre, Ziyu Wang, Tobias Pfaff, Yuhuai Wu, Roman Ring, Dani Yogatama, Dario Wünsch, Katrina McKinney, Oliver Smith, Tom Schaul, Timothy Lillicrap, Koray Kavukcuoglu, Demis Hassabis, Chris Apps & David Silver, "Grandmaster level in StarCraft II using multi-agent reinforcement learning", Nature volume 575, pp. 350–354 (2019) ↩︎
Author Bio
Gary Marcus is a scientist, best-selling author, and entrepreneur. He is the founder and CEO of Robust.AI, and was the founder and CEO of Geometric Intelligence, a machine learning company acquired by Uber in 2016. He is the author of five books, including The Algebraic Mind Kluge, The Birth of the Mind, and The New York Times best seller Guitar Zero, and his most recent book, co-authored with Ernest Davis: Rebooting AI.
Acknowledgments
Thanks to Paul Tune, Justin Landay, and Hugh Zhang for their help with editing this piece.
Citation
For attribution in academic contexts or books, please cite this work as
Gary Marcus, "An Epidemic of AI Misinformation", The Gradient, 2019.
BibTeX citation:
@article{marcus2019hype,
author = {Marcus, Gary},
title = {An Epidemic of AI Misinformation},
journal = {The Gradient},
year = {2019},
howpublished = {\url{https://thegradient.pub/an-epidemic-of-ai-misinformation/ } },
}
If you enjoyed this piece and want to hear more, subscribe to the Gradient and follow us on Twitter.