Surveillance is becoming an increasingly controversial application given the rapid pace at which AI systems are being developed and deployed worldwide.
While protestors marched through the city demanding justice for George Floyd and an end to police brutality, Minneapolis police trained surveillance tools to identify them. With just hours to sift through thousands of CCTV camera feeds and other dragnet data streams, the police turned to a range of automated systems for help, reaching for information collected by automated license plate readers, CCTV-video analysis software, open-source geolocation tools, and Clearview AI’s controversial facial recognition system. High above the city, an unarmed Predator drone flew in circles, outfitted with a specialized camera first pioneered by the police in Baltimore that is capable of identifying individuals from 10,000 feet in the air, providing real-time surveillance of protestors across the city. But Minneapolis is not an isolated case of excessive policing and technology run amok. Instead, it is part of a larger strategy by the state, local, and federal government to build surveillance dragnets that pull in people’s emails, texts, bank records, and smartphone location as well as their faces, movements, and physical whereabouts to equip law enforcement with unprecedented tools to search for and identify Americans without a warrant.
The federal government has worked hand in glove with state and local police to create the kind of pervasive and precise surveillance systems that the Founding Fathers explicitly sought to reject with the Fourth Amendment. Explicitly written to tie the police powers of the state, the Fourth Amendment grew out of the colonists’ experience with agents of the British Crown who, in an effort to stamp out rampant smuggling in the colonies, used “writs of assistance” to search any American and confiscate his or her important documents and possessions without a warrant or even an arrest. The Fourth Amendment forces the government to produce evidence that a suspect committed a crime (called probable cause) and a warrant signed by a judge stating what documents or information they believe they’ll find when they search him or her. However, those protections have eroded in the digital age. Technology and lax data and privacy laws have enabled the rise of dragnet surveillance systems that regularly search and seize critical data and devices from Americans without a warrant or a crime committed, relying on automated systems to carry out modern-day, digital writs of assistance on Americans.
Surveillance: It's Here Already
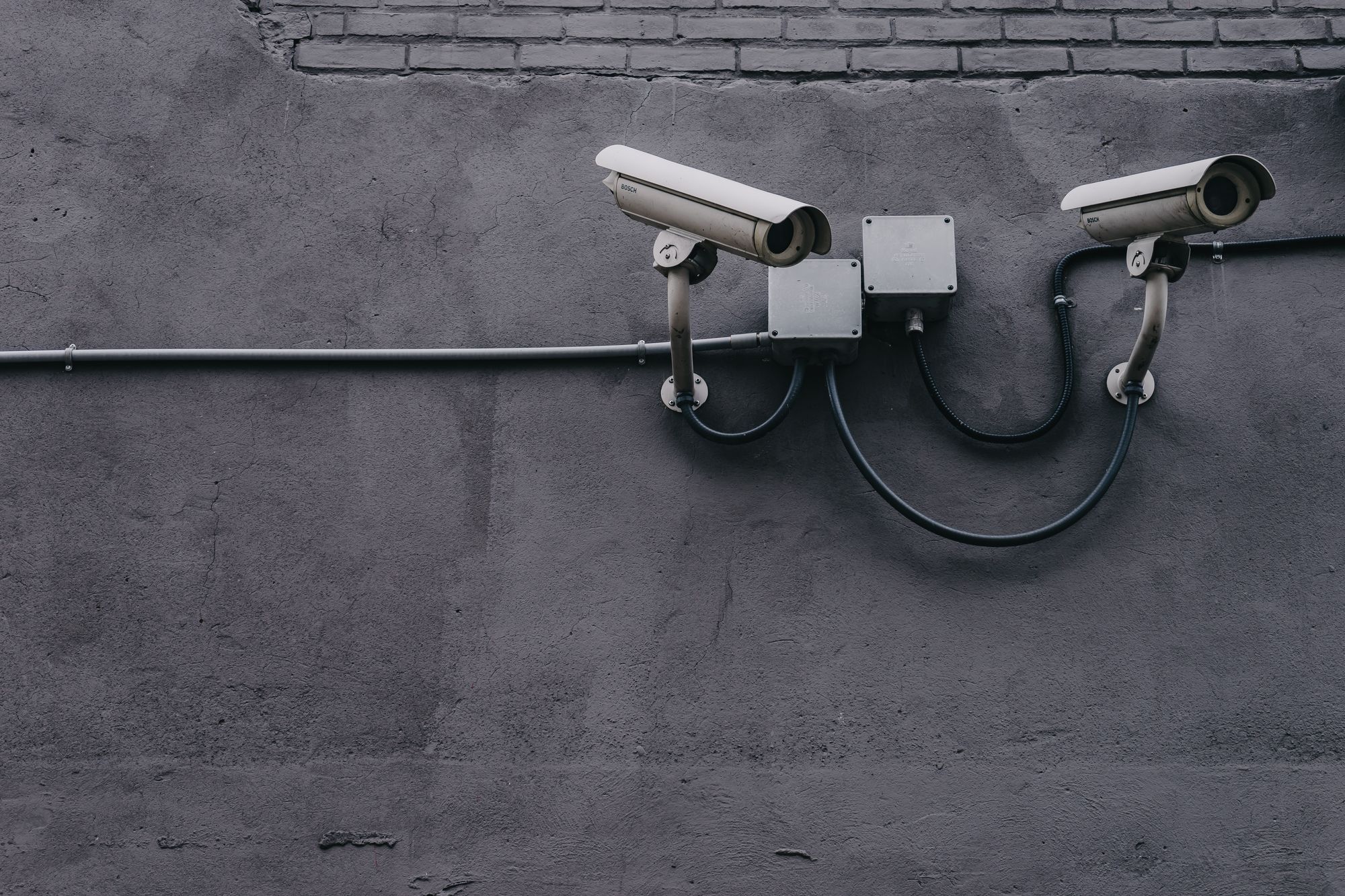
How Police use Machine Learning
Like in Minneapolis, state and local police all over the US use a range of automated tools, including facial recognition, license plate readers, StingRays (cell-site simulators), predictive policing, video analysis tools and more, to create advanced systems of surveillance with little oversight, all built largely out of the public’s view despite their reach into the lives of ordinary Americans. In Miami, a protestor who allegedly threw something at police officers was later identified and arrested using Clearview AI’s facial recognition system, although the police report makes no mention of using the technology. In Baltimore, the police relied on StingRays, facial-recognition software linked to the city’s CCTV cameras, and a surveillance drone capable of identifying individuals from more than 10,000 feet above the city to monitor the Freddie Gray protests in real-time. In 2018, in response to more protests and riots, Baltimore police used facial recognition through the city's CCTV camera network to identify and later arrest protestors. Similarly, the New York Police Department arrested more than 3,000 people using facial recognition technology in the first five-and-a-half years of the program. Surveillance drones first flown by the FBI during the Freddie Gray protests continue to prowl Baltimore’s skies to this day. A private contractor blandly called Persistent Surveillance Systems (PSS) has been flying a surveillance drone over the city since 2016 and claims the footage can be linked with the city’s CCTV cameras to spot crime and identify the suspects in real-time. “From a plane flying overhead, powerful cameras capture aerial images of the entire city. Photos are snapped every second, and the plane can be circling the city for up to 10 hours a day.” According to the ACLU, the pervasive and precise surveillance system built in Baltimore became the “technological equivalent of putting an ankle GPS [Global Positioning Service] monitor on every person in Baltimore.” When reports about the program surfaced, Baltimore shuttered the program in 2016. But, in April of this year, the city reversed course and awarded PSS a contract to fly three surveillance drones over Baltimore again, with the blessing of a district court judge.
Researchers would like to think that their work in AI or robotics can be separated from politics of the moment. They are wrong. Without the call and funding for the advancement of AI in critical areas such as biometric recognition and big data analysis by law enforcement, researchers at Microsoft, Amazon, and Palantir would not be at the cutting-edge of surveillance systems. Recent reports shed light on the extensive network of surveillance tools, automated systems, and databases police departments have built that rely on research and technology from commercial partners to fuse and analyze criminal records, city-wide cameras systems, and a firehose of data about a person’s on-and offline life. For example, in New York City, Microsoft has worked closely with the NYPD to develop a city-wide surveillance platform called Domain Awareness System (DAS) since 2009. Details about the platform are only now coming to light DAS appears to be the most comprehensive surveillance system in a major US city to date. The system draws in data to deliver three core functions: real-time alerting, investigations, and analytics. Similar to Alibaba’s “city-brain” projects, DAS expands data collection and centralizes data analysis into an integrated platform, creating a digital perch from which the police can watch the entire city.
According to The Intercept, which broke the story and obtained slides from Microsoft and the NYPD detailing the DAS platform, the system first ingested information from CCTV cameras, environmental sensors (e.g., to detect radiation), and automated license plate readers. The network, however, quickly expanded, relying on automated systems to reach deeper into the city. “By 2010, it began adding geocoded NYPD records of complaints, arrests, 911 calls, and warrants ‘to give context to the sensor data.’ Thereafter, it added video analytics, automatic pattern recognition, predictive policing, and a mobile app for cops. By 2016, the system had ingested 2 billion license plate images from ALPR cameras (3 million reads per day, archived for five years), 15 million complaints, more than 33 billion public records, over 9,000 NYPD and privately operated camera feeds, videos from 20,000-plus body cameras, and more. To make sense of it all, analytics algorithms pick out relevant data, including for predictive policing.” For more than a decade, Microsoft and the NYPD have been creating a city-wide surveillance platform that fuses multiple channels of both real-time and historical data with AI to process and make sense of it all. Automated tools of data collection and analysis work shoulder to shoulder with local and national law enforcement to extend their reach in cities across the US.
However, the surveillance systems built by police in New York, Baltimore, and Minneapolis are not isolated examples of excessive policing in a few metropolitan police departments, but are part of a larger pattern of an expanding surveillance apparatus that is granting law enforcement unprecedented powers to watch, search, and seize the sensitive data of American citizens without a warrant or even suspicion of a crime. Even getting a count of which police departments use what tools is difficult because many of these programs are shrouded in secrecy and details come to light from media reports after the fact. The most complete accounting to date was recently compiled by the Electronic Frontier Foundation (EFF), which combed through thousands of public records to build an “Atlas of Surveillance.” The map offers the most detailed look at the wide variety of automated surveillance tools at the disposal of state and local law enforcement all across the country. Some of the numbers are eye-popping: at least 1074 jurisdictions and/or police departments use drones; 360 use facial recognition; 64 use StingRays; 24 use video analysis and/or computer vision tools (automated data analysis tools); and 26 employ predictive policing measures.
In a new twist, these surveillance systems are starting to seep out of metropolitan police departments and into the suburbs. According to the EFF, 1,328 police departments have partnerships with Amazon’s Ring home security camera system, giving police access--and in some cases live access--to recordings from private property without getting a warrant first. Everyday citizens and neighborhood watch programs are working hand in hand with local and national law enforcement to build a similarly pervasive and precise surveillance system outside the city as well. These Ring partnerships lay bare the incentives of private industry and law enforcement align to drive the explosive growth of the surveillance industry. For example, the global video surveillance market is expected to reach \$144 billion by 2027 from about \$40 billion in 2019. As the Wall Street Journal noted, the surveillance industry grew from practically “$0” in 2001 to the multibillion-dollar global market it is today. In pursuit of more data and digital eyes and ears on its citizens, the US government is among the market’s biggest players: although estimates of the cost of government surveillance programs are difficult to make, in 2016 the Washington Post reported that the FBI’s budget included \$600-\$800 million for its Office of Advanced Technology to develop high-tech surveillance tools, including how to break all forms of digital encryption.
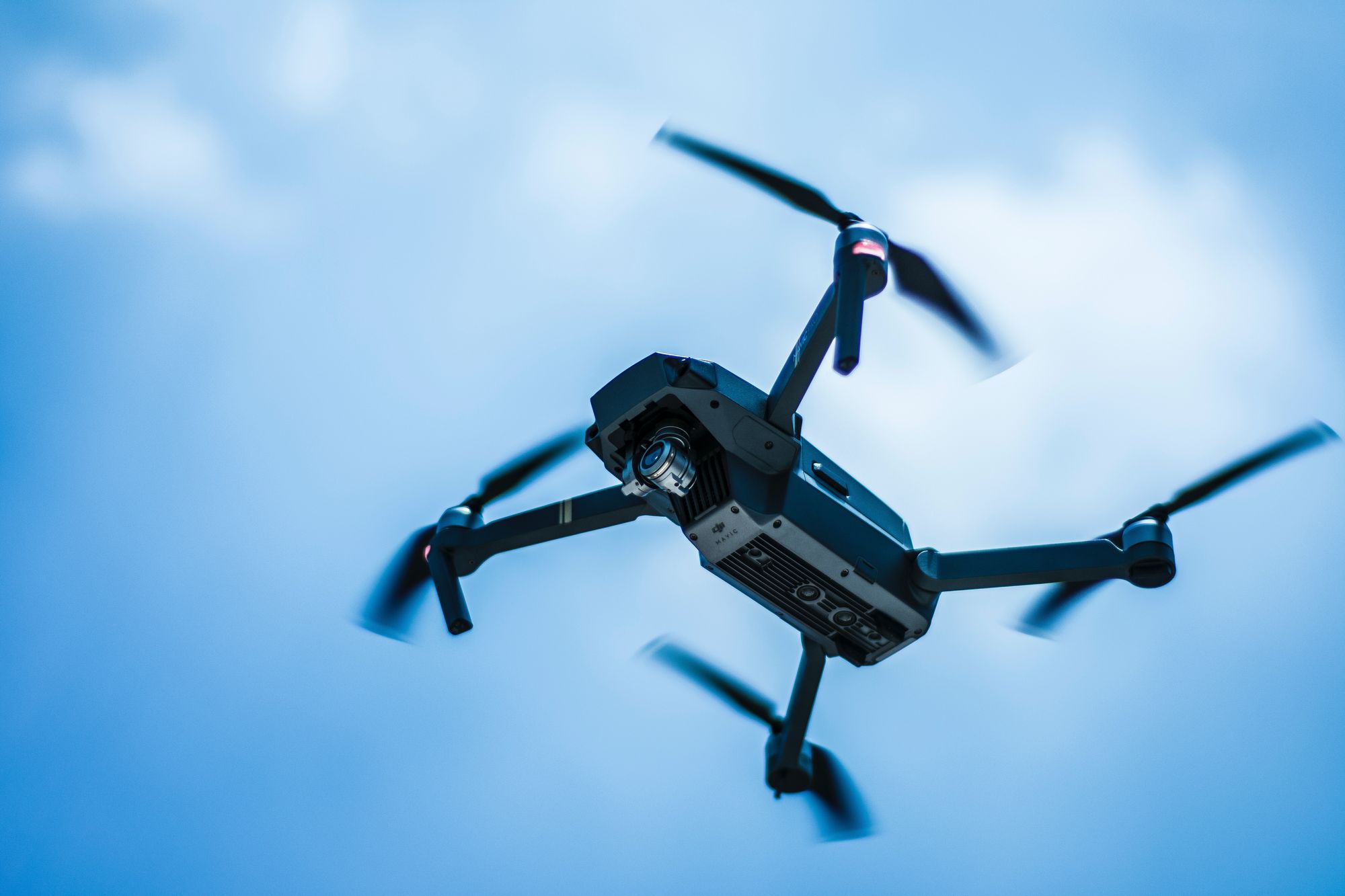
How the Government uses Machine Learning
Like law enforcement in New York, the US government is amassing an unprecedented amount of information about its citizens using an array of automated surveillance tools. Lacking legislation from Congress unifying the states’ approaches into a national framework to guide data collection, America’s privacy and data laws are a patchwork of incomplete and competing rules, and offer little restraint or oversight over the police’s collection of personal information (the exception being Illinois, the first state to pass a law requiring consent to collect biometric data, which was expansively defined to include fingerprints, face scans, iris scans, and more, and that the data be deleted at a later time). On top of the continuous streams of data coming in from tools like automated license plate readers or CCTV cameras, law enforcement maintains a variety of databases, many of which now depend on automated collection and processing to glean insights from the sheer volume of data the police now ingest. State, local, and national police have access to more than 20 databases that cover a person's criminal history and his or her interactions with the state (e.g., drivers licenses) to build a “pattern of life” of the targeted individual, who may or may not have committed a crime.
To get an idea of the amount of information the government has collected on Americans, take the National Crime Identification Center (NCIC), the FBI’s centralized crime database. These records include such things as the content of communications such as phone calls and emails; medical diagnoses, treatments, and conditions; Internet browsings; financial transactions; physical locations; bookstore and library purchases, loans, and browsings; other store purchases and browsings; and media viewing preferences. Both local and federal law enforcement also maintain biometric databases, including those containing blood samples, fingerprints, facial recognition, and DNA samples (in addition to those maintained by police, consumer genetics databases such as GEDMatch are proving invaluable sources of information, like in the Golden State Killer case). Few controls or oversight exist to constrain how the police use and share the information once it is collected.
Once the government has obtained your information legally (and sometimes illegally), that data can be shuttled between a variety of state, local, and federal agencies. At the national level, fusion centers serve as the link between local and federal police so that data and intelligence tools can legally flow in both directions. Although they are young, another post-9/11 creation, the government already operates 76 such centers across the country. Fusion centers receive information from a variety of sources--local, state, and federal law enforcement as well as homeland security partners and private entities--functioning as, “regional focal points for gathering and sharing government and private information related to ‘threats.’” Indeed, DHS emphasizes that this is not the federal government intruding into state and local affairs, but that, “In recent years, partners at all levels of government have reiterated the need for unified and coordinated support for fusion centers.” Though decentralized and diffuse, fusion centers are the focal points of a growing, mass surveillance network that connects state and local police with the tools and data intelligence of the national spy agencies. Law enforcement at all levels of government share information to expand the total pool available to them without little oversight or transparency about how the data is used. For example, the state of Maryland gave the FBI access to its databases of 7 million drivers license photos and 3 million mugshots, leaving 10 million Marylanders open to unchecked facial recognition searches by federal agents. Once in the system, you are in a perpetual line-up, always a potential suspect. The Fourth Amendment sets a high legal bar for law enforcement to search you, requiring probable cause and a warrant for the government to access your, “persons, houses, papers, and effects.” However, modern technology has carved out loopholes in the Fourth Amendment that enable the kind of pervasive and precise surveillance the Founders meant to prevent with the Bill of Rights.
Predictive Policing
Predictive policing programs are another illustrative example showing how data, surveillance technology, and a system of automated policing work together to spy on, search, and, ultimately, control Americans who have not committed or been convicted of a crime. Predictive policing is premised on the idea that historical data of crime, demographics, socioeconomics, and geography can be used to forecast future incidents. Knowing where crime is likely to occur again, police try to intervene beforehand and prevent it. Broadly there are two kinds of “heat maps” produced by predictive policing models: place-based, which uses less data to try to avoid systemic pitfalls of relying on crime and demographic data and surges police into specific areas, and person-based, which tracks and creates a list of “high-risk” individuals by combining a person's criminal history with an analysis of their social network. However, most predictive policing programs are systemically racist in operation in large part because the data they are fed are biased to reflect real world racial inequalities. Although it is frequently billed as “crime data,” the data that most policing algorithms are built on is in fact arrest data, which is further biased because people of color are arrested at higher rates than white people despite committing similar rates of crime. Predictive policing programs that depend on crime or other historical data inevitably develop serious blind spots and often reproduce the very prejudices of the criminal justice system that AI was brought in to address.
Despite concerns about structural bias and overpolicing, predictive policing programs are spreading--the Atlas of Surveillance pinpoints 26 jurisdictions that use predictive policing today. Chicago ran one of the most comprehensive and studied predictive policing systems in the country from 2012, until it shuttered the program in January of this year after facing public pressure over how intrusive and ineffective the system was. Inspired by an epidemiological model of violent crime developed by Yale researchers, the Chicago Police Department (CPD) program aimed to identify high-risk individuals that were vectors of violent crime. The algorithm drew upon criminal and historical data to produce maps that would show where future crimes were likely to occur and data and paired with social network analysis to create a “strategic short list” of those most likely to shoot someone or be shot themselves. Tracing the course of violent crime requires gathering information about the history or geography of crime in any city as well as identifying specific people. That’s a massive amount of data in both aggregate and granular form that law enforcement is feeding into predictive policing algorithms. Tracking what data is collected, where it is stored, or who has access to it can be difficult, even for the city government. For example, police used a similar predictive policing model based in New Orleans, relying on social network analysis to draw connections, “people, places, cars, weapons, addresses, social media posts, and other indicia in previously siloed databases…. After entering a query term — like a partial license plate, nickname, address, phone number, or social media handle or post — NOPD’s analyst would review the information scraped by Palantir’s software and determine which individuals are at the greatest risk of either committing violence or becoming a victim, based on their connection to known victims or assailants.” City council members found out about the program after reading about it in the newspapers.
In 2013 the researchers from the RAND Corporation, a social science think tank, were granted unprecedented access to the CPD’s predictive policing program, sitting in on strategy meetings and riding with officers for months. Despite the technological sophistication of the tools used, the RAND researchers study concluded that the predictive policing program, “was ineffective, and a legal battle revealed that the list, far from being narrowly targeted, included every single person arrested or fingerprinted in Chicago since 2013.” In fact, the researchers found predictive policing led to no significant difference in murder or violent crime rates. Rather than informing a system focused on preventing and mitigating crime, the list was used to target people for arrests. Although predictive policing programs have been shown to be ineffective and systemically discriminatory, their use is rapidly spreading in cities across the US. In response, some cities such as San Francisco, Boston, and Portland have targeted pieces of the police’s dragnet surveillance system, banning the use of facial recognition by the public authorities. But in most of the country, these tools are largely unregulated by the legislature, constrained by lax use of force laws and policy becomes how the government uses the technology. In the hands of law enforcement, these extraordinary powers of search and seizure are trained towards arresting people rather than preventing crime.
Given the dramatic expansion of surveillance by local and national law enforcement, Congress has considered a series of bills that would rein in biometric recognition programs and establish an oversight mechanism into these surveillance systems. Last month, Senators Ed Markey (D-MA) and Jeff Merkley (D-OR) introduced legislation that would ban the use of facial and other biometric recognition technology by federal agencies as well as make federal and state funding for law enforcement contingent on enacting similar bans. Senator Merkley also introduced the Algorithmic Accountability Act with Senator Cory Booker in February, which would direct the Federal Trade Commission to write regulations for companies under its jurisdiction to study and correct their algorithms if they make an inaccurate, biased, or discriminatory decision impacting Americans. Both are unlikely to pass. Without a framework from Congress, however, the public is left in the dark trying to restore the surveillance powers of the state to the balance the Founders intended.
Written to tie the hands of the state, the Fourth Amendment of the US constitution protects citizens from unreasonable searches and seizures without probable cause and a warrant that lays out specifically what the police think they might find. But technology has opened the door to mass surveillance, enabling governments to build the very dragnet systems that give the government a picture of a person’s “pattern of life” without a warrant or probable cause. Last year, it was revealed that Google is storing detailed location history on millions of people in its “Sensorvault,” which allowed those with access to see the location history of anyone with an Android smartphone or Google Maps installed on their phone within a specific time and place. Police at all levels of government have been eager to add Sensorvault to their investigative toolkit. Google reported processing 180 of these “geo-fencing” warrants a week for the FBI and police departments in North Carolina, California, Florida, Maine, and Minnesota (and those are just the ones we know about). As the EFF points out, police do not name a suspect or even a target device in their geo-fenced warrants, instead working backward from a specific time and place where a crime occured. And yet, as the Supreme Court noted in 2018, this kind of travel data can be used to paint a detailed portrait of the target’s life, providing, “an intimate window into a person's life, revealing not only his particular movements, but through them his ‘familial, political, professional, religious, and sexual associations.’” Without naming a suspect or targeting a specific device, geo-fence warrants allow the police to cast a dragnet that ensnares anyone in a particular time and place. This makes it a fishing expedition--the very kind of generalized searches and seizures the 4th Amendment was meant to prevent.
The post-9/11 national security surge has built an intrusive surveillance state that conducts the kind of pervasive and precise dragnet of searches and seizures the Fourth Amendment was written to prevent. Aided by automated technology, law enforcement at all levels of government have amassed an unprecedented amount of information about Americans, many of whom have committed no crime. Using facial recognition, automated license plate readers, StingRays, unarmed Predator drones, geofenced warrants accessing Google’s SensorVault, and geolocation from people’s social media accounts and smartphones, police across the country regularly spy on and identify American citizens with little pretext beyond the invisible security state’s suspicion of a crime. Together, technology and law enforcement have hollowed out the 4th amendment, creating the very precise and pervasive surveillance system the Bill of Rights was written to protect. The dangers of an invisible and omnipresent security state are becoming increasingly apparent. Last month, it was revealed the Department of Homeland Security authorized the domestic surveillance of protestors and journalists, training a system usually reserved for hunting terrorists overseas with drones on American citizens exercising their First Amendment rights. DHS went as far as to create intelligence reports on a journalist from the New York Times and a legal expert for the national security law blog “Lawfare.” These are just a few incidents (that we know of), but they should serve as stark warnings that the overbuilt surveillance state may be slipping out of the public’s control.
Protests continue to roil cities across America as protestors and police meet in increasingly confrontational ways. A heated spring gave way to a hot and violent summer. In city after city, from Portland and New York City down to tiny Kenosha, Wisconsin, police responded to these protests--which are now recognized as one of the largest mass social movements in American history--by cracking down on the protestors and clearing the streets by force. Less easily spotted, amid the pandemonium, were the automated systems law enforcement rely on to coordinate their response to the protests, spy on them, identify them, and later locate them for arrest. But police could be confident in the dragnet surveillance systems being built across the country to help them spy on and target protestors and rioters. Whether by drone circling 10,00 feet above the city, facial-recognition algorithms behind every CCTV camera lens, a replay of your smartphone location history in Google’s sprawling SensorVault, the police have no shortage of options to turn to if they want to locate and identify you.
Author Bio
Bryan McMahon is an AI Policy Researcher at NEDO, the Japanese government’s science and technology R&D organization, where he analyzes US and international AI policy strategies. He earned a B.A. in Biology from Duke University, where he studied science policy in its Science and Society Program, a research institute sitting at the intersections of science, law, and policy. Previously, his work on artificial intelligence policy has been published in the Journal of Science Policy and Governance as well as a recent piece analyzing the rules governing so-called ‘killer robots’ in Skynet Today.
Acknowledgments
The main image from this piece is a photo by Francesco Ungaro from Pexels.
Citation
For attribution in academic contexts or books, please cite this work as
Bryan McMahon, "How the Police Use AI to Track and Identify You", The Gradient, 2020.
BibTeX citation:
@article{
mcmahon2020surveillance,
author = {McMahon, Bryan},
title = {How the Police Use AI to Track and Identify You},
journal = {The Gradient},
year = {2020},
howpublished = {\url{https://thegradient.pub/how-the-police-use-ai-to-track-and-identify-you/ } },
}
If you enjoyed this piece and want to hear more, subscribe to the Gradient and follow us on Twitter.