Every day we hear claims that Artificial Intelligence (AI) systems are about to transform the economy, creating mass unemployment and vast monopolies. But what do professional economists think about this?
Economists have been studying the relationship between technological change, productivity and employment since the beginning of the discipline with Adam Smith’s pin factory. It should therefore not come as a surprise that AI systems able to behave appropriately in a growing number of situations - from driving cars to detecting tumours in medical scans - have caught their attention.
In September 2017, a group of distinguished economists gathered in Toronto to set out a research agenda for the Economics of Artificial Intelligence (AI). They covered questions such as what is economically unique about AI, what will be its impacts, and what are the right policies to spread its benefits.
Last September I had the privilege of attending the third edition of this conference in Toronto, and to witness first-hand how the Economics of AI agenda has evolved. Here, I outline the key themes of the conference and relevant papers at four levels:
- Macro View: Impact of AI on aggregate economic variables like productivity, employment or inequality
- Meso View: Impact of AI on individual sectors such as scientific research or regulation
- Micro View: Impact of AI on the behaviors of organizations and individuals
- Meta View: Impact of AI on the data and methods that economists use to study AI
I then outline some gaps in today's Economics of AI agenda to be addressed in future research.
An economist's take on AI
Ajay Agrawal, Joshua Gans and Avi Goldfarb, the convenors of the conference (together with Catherine Tucker), have in previous work described AI systems as "prediction machines" that make predictions cheap and abundant, enabling organizations to make more and better decisions, and automating some of them. One example of this is Amazon's recommendation engine, which presents a personalized version of its website to each visitor. That kind of customization would not be possible without a machine learning system (a type of AI) that predicts automatically what products might be of interest to the individual customer based on data about her behavior and other customers who are similar to her.
AI systems could be adopted by any sector facing a prediction problem - which is almost anywhere in the economy from agriculture to finance. This widespread relevance of AI has led some economists to herald it as the latest example of a transformational "General Purpose Technology" that will reshape the economy like the steam engine or the semiconductor did earlier in history.
The Macro View
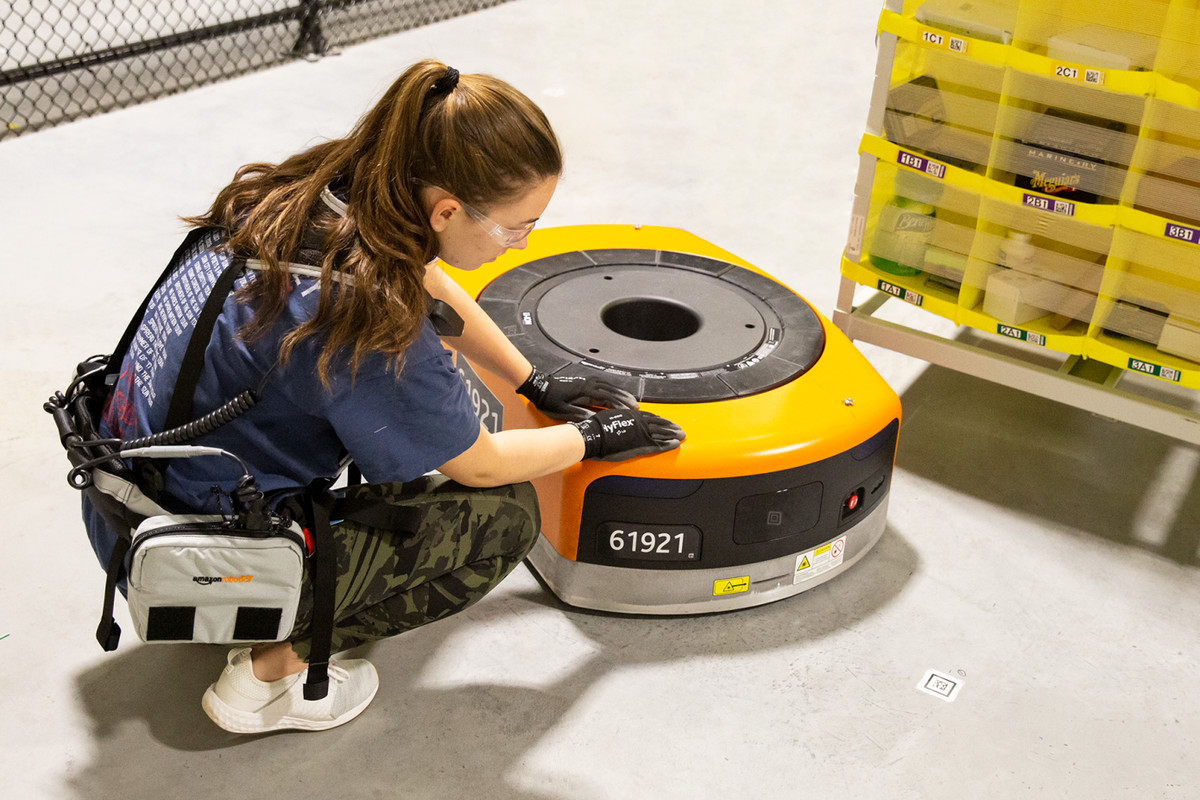
AI automates and augments decisions in the economy, and this increases productivity. What are the implications for labor and investment?
The task-based model
The main framework to analyze the impact of AI on labor is the task-based model developed by Daron Acemoglu and Pascual Restrepo (building on previous work by Joseph Zeira). This model conceives the economy as a big collection of productive tasks. The arrival of AI systems able to perform some of these tasks impacts on demand for labor, the share of income that goes to it (or to capital), and inequality. For example, if AI de-skills labor or increases the share of income going to capital - which tends to be concentrated in fewer hands - this is likely to make our economy more unequal.
The impact of AI on tasks happens through four channels:
- First, there is displacement, when an AI system replaces some of the tasks that were previously performed by humans. An example of this would be the book reviews that were displaced when Amazon adopted its automatic recommender (and laid off its book reviewers, although some have now made it back to the company). This will reduce demand for labor.
- Second, there is augmentation when an AI system increases the value of the tasks carried out by humans. An example of this would be Amazon's web development and inventory management tasks: each dollar spent improving its website and stocking many different titles creates a bigger return for the company thanks to its AI recommendation system. This will in general increase the demand for workers whose tasks are augmented.
- Third, there is capital deepening. New AI systems are an investment that increases the stock of capital that workers use, making them more productive and increasing demand for labor through the same mechanism as above.
- Finally, there is reinstatement, when the AI system creates completely new tasks such as developing machine learning systems or labeling datasets to train those systems. These new tasks will create new jobs and even industries, increasing labor demand.
Considered together, these four channels determine the impact of AI on labor demand. Contrary to the idea of the impending job apocalypse, this model identifies some channels through which AI systems could increase demand for labor. At the same time, and contrary to an standard assumption in economics that new technologies always increase labor demand through augmentation, the task-based model recognizes that the net effect of new technology on labor demand could be negative. This could, for example, happen if firms adopt "mediocre" AI systems that are productive enough to displace workers, but not productive enough to increase labor demand through the other channels.
Several papers presented in the conference developed these themes:
- Jackson and Kanik model AI as an intermediate input that firms acquire through their supply chain using services such as Amazon's Web Services. In this model, the impact of AI on labor demand and productivity depends on the alternative employment options for those workers who are displaced by AI: if alternative jobs have low productivity, then AI will have an (indirectly) negative impact on productivity. This means that the impacts of AI depend not only on what happens in AI adopting sectors but also on the situation elsewhere in the economy. Another interesting conclusion of this analysis is that AI deployment makes the economy more interconnected as companies start using AI suppliers to source services previously done by workers. This could centralize value chains, increasing market power and creating systemic risks.
- Autor and Salomons study the evolution of the industries and occupations that create new job titles (a proxy for new tasks) using a dictionary of job titles published by the US Census since the 1950s. Their analysis shows important changes between then, when occupations in the middle of the income distribution ("middle-class jobs") created most new job titles, and now, when most of the new job titles are created in either highly-skilled, technology-intensive occupations (eg. software development) or less skilled personal services occupations (eg. personal trainers). It looks like modern technologies such as AI increase demand for high skilled jobs that complement AI and low-skill jobs that are difficult to replace with AI, leading to polarization in the labor market. There is also the risk that skills shortages in highly-skilled occupations may coexist with unemployment amongst individuals lacking the skills to transition into those occupations.
Automation without capital
In order to increase productivity, investments in AI needs to be accompanied by complementary investments in IT infrastructure, skills and business processes. Some of these investments involve the accumulation of "intangibles" such as data, information and knowledge. In contrast to tangible assets like machines or buildings, intangibles are hard to protect, imitate and sell, and their creation often involves costly experiments and learning by doing (much more on this subject here).
Continuing with the example of Amazon, over its history the company has built a tangible data and IT infrastructure complementing its AI systems. At the same time, it has developed intangible processes, practices and a mindset of "customer-centrism" and open interfacing between its information systems and those of its vendors and users that is perhaps equally important for its success, but at the same time very hard to imitate.
According to a 2018 paper by Erik Brynjolfsson and colleagues, the need to accumulate these intangibles across the economy could explain why advances in AI are taking so long to result in productivity growth or drastic changes in labor demand.
Several papers presented in Toronto this year explored these questions empirically:
-
Daniel Rock uses LinkedIn skills data to measure the impact of engineering skills on firm value. He finds that after controlling for unobservable firm factors, the link between those skills and firm value dissipate, suggesting that intangible firm factors determine the business impact of engineering talent. His analysis also suggests that the market expects these intangible investments to generate important returns in the future: when Google released TensorFlow, those firms already employing AI talent experienced an increase in their market value. One explanation is that inventors saw TensorFlow as a tool that would help those firms create value from their intangible AI-related investments. Interestingly, similar increases in market value were not visible in firms whose workforces were at risk of automation. One interpretation is that these firms are expected to be disrupted by the developers of AI systems and services.
-
Prasanna Tambe and co-authors also use LinkedIn data to estimate the value of intangible investments related to AI, finding that it is concentrated in a small group of "superstar firms", and that it is associated with higher market value This means that the market expects the benefits from AI to be concentrated in a few firms, raising concerns about market power in tomorrow's AI-powered economy.
Differences in AI adoption and impacts
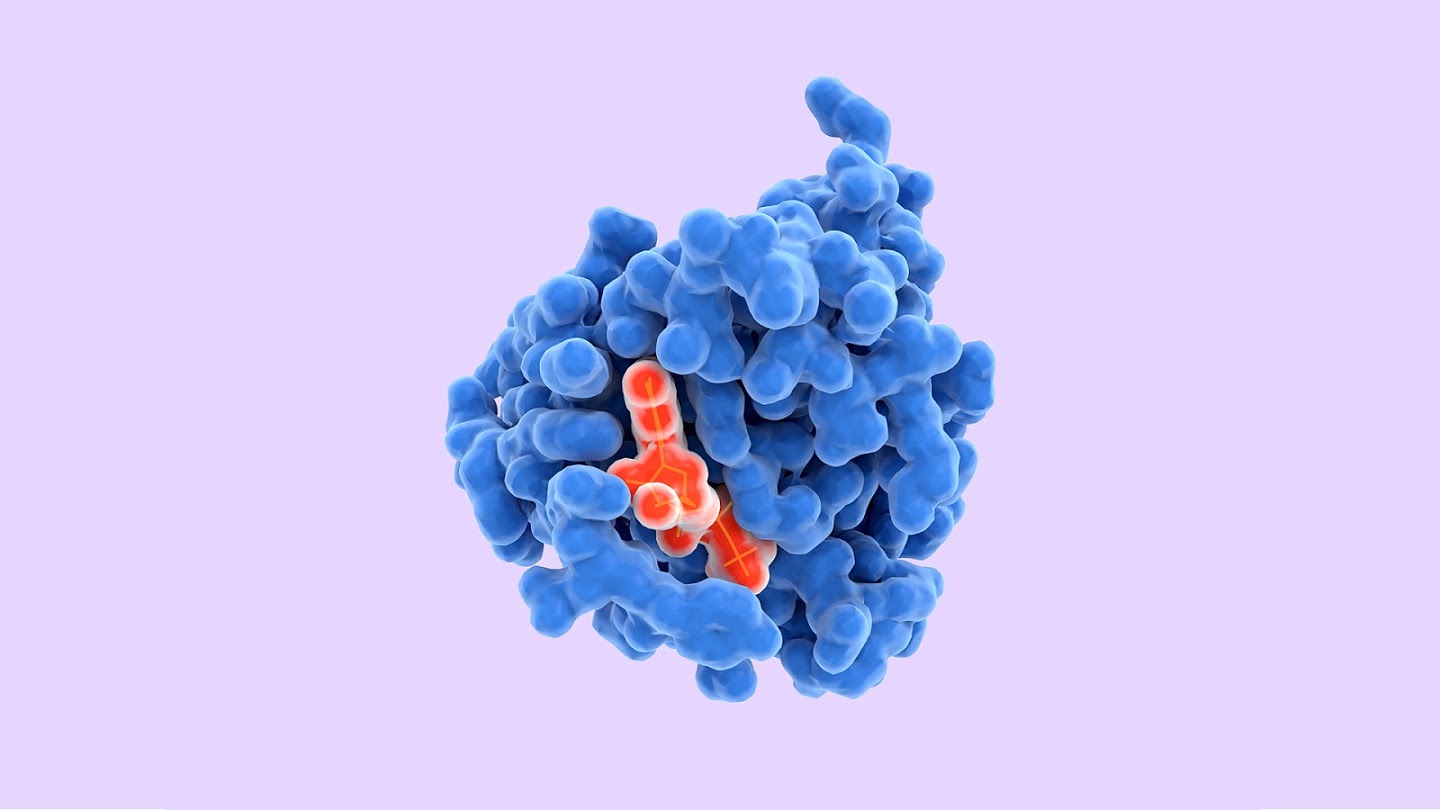
Think of a sector like health: the nature of production in this industry, as well as the availability of data, the scope to change business processes and its industrial structure (including levels of competition and entrepreneurship), are completely different from, say, finance or advertising. This means that AI will have a very different impact there from what happens in other industries.
Previous editions of the Economics of AI conference included papers about the impact of AI in sectors such as media or health-care. This year considered sector-specific issues in several areas including scientific R&D and regulation.
Sending machines to look for good ideas
In the inaugural Economics of AI conference, Cockburn, Henderson and Stern proposed that AI is not just a General Purpose Technology, but also an "invention in the methods of invention" that could transform the productivity of scientific R&D, generating important spillovers in the sectors using that knowledge. One could even argue that the idea of the Singularity is an extreme case of this model where "AI systems that create better ideas" become better at creating "AI systems that create better ideas" in a recursive loop that leads to exponential growth.
This year, venture capitalist Steve Jurvetson and Abraham Heifts, CEO of Atomwise, a startup that uses AI in drug discovery spoke about how they are already pursuing some of these opportunities in their ventures. Two papers investigated the impact of AI on R&D:
- Analysis by myself and colleagues of the deployment of AI in computer science research in arXiv supports the idea that AI is, at least, an invention in the methods of computing: AI activity has seen rapid growth in absolute and relative terms, it is being adopted in many computer science subfields, and it is already creating important impacts (measured with citations) wherever it is adopted. AI is being taken up faster in fields such as computer vision, natural language processing, sound processing and information retrieval where there are big datasets to train machine learning systems, highlighting how AI R&D advances faster in those areas with lots of data
- Agrawal and co-authors develop a formal model of the impact of AI on the R&D process in scientific fields such as bio-medical and materials science, where innovation often involves finding useful needles in big data haystacks. An example of this is identifying which, among the millions of potential folds in a protein could be targeted by a pharmaceutical drug. AI systems could help identify which of these combinations have the greatest potential, reducing waste and reviving productivity growth in R&D. According to the authors, realizing these benefits will require access to training data and building research teams that bring together AI skills with domain knowledge about the scientific fields where AI is being adopted.
AI Regulation
Regulation sets the rules of the game for the development and adoption of new technologies like AI . At the same time, regulation is itself an industry whose structure and processes are being transformed by AI systems that speed up technology change and create new opportunities to monitor economic activity. Two talks at the conference focused on this two-way street between regulation and AI.
- Suk Lee and co-authors have surveyed businesses about how they would change their AI adoption plans in response to different models for AI regulation. They show that general-purpose regulations would create more barriers to AI adoption than sector-specific regulations, and that regulation increases demand for managers to oversee AI adoption while reducing demand for technical and lower-skilled workers. It also creates bigger barriers for smaller firms, highlighting the potential costs of tighter regulation in terms of innovation and competition.
- Clark and Hadfield argue that the regulatory industry needs to be innovative to keep up with the fast pace of change in AI technologies, but public-sector regulators lack flexibility and incentives to do this effectively. To address this, they propose the creation of regulatory markets where the government licenses private companies to regulate AI adoption with measurable goals (for example to lower AI error rates and accidents below an agreed threshold): this would give private sector firms the incentives and freedom to develop innovative regulatory technologies and business models, although it also raises the question of who would regulate these new regulators, and how to avoid their capture by the industries they are meant to watch over.
Micro view
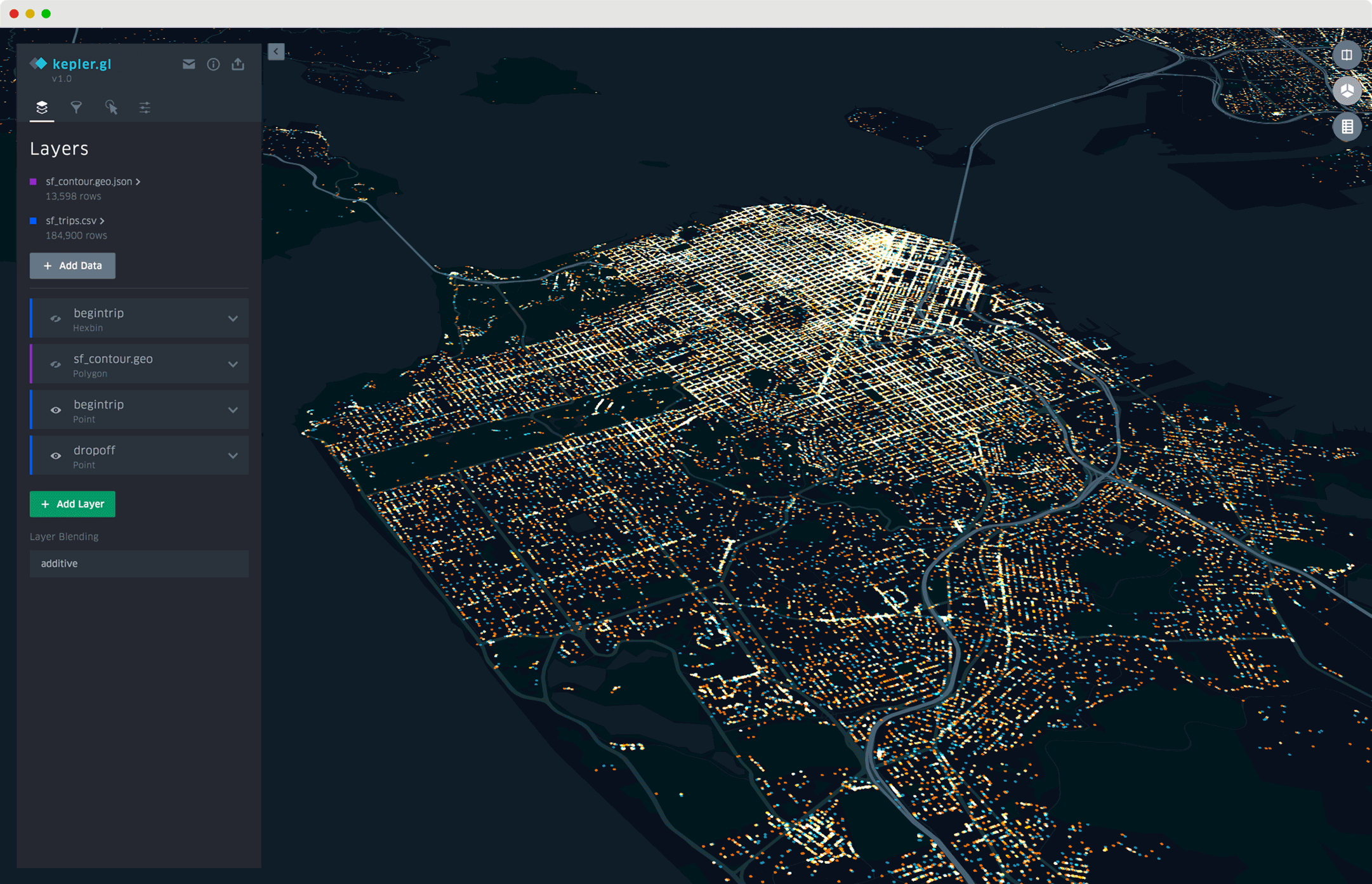
Modern AI systems based on machine learning algorithms that detect patterns in data are often referred to as black boxes because their predictions are hard to explain and understand. Similarly, the firms adopting AI systems look like black boxes to economists adopting a macro perspective: AI intangibles are after all a broad category of business investments including experiments with various processes, practices and new businesses and organizational models. But what are these firms actually doing when they adopt an AI system, and what are the impacts?
Several papers presented at the conference illustrated how economists are starting to open these organizational black boxes to measure the impact of AI. As they do this, they are also incorporating into the Economics of AI some of the complex factors that come into play when firms deploy AI systems that do not just increase the supply of predictions, but also reshape the environment where other actors (employees, consumers, competitors, the AI systems themselves) make decisions, leading to strategic behaviors and unintended consequences.
- Susan Athey and co-authors compare the service quality of UberX and UberTaxi rides in Chicago. They confirm the hypothesis that UberX drivers whose job depends on user reviews will provide higher quality rides with an analysis of detailed telematics data about driving speed and duration, number of hard brakes etc. They also test whether giving drivers information about their performance changes their behavior, finding that the worst performers tend to improve their driving in response to these "nudges". The paper shows that AI systems are an "invention in the methods of managing and regulating increasingly important digital platforms and marketplaces", while also raising substantial concerns about worker privacy and manipulation.
- Michael Luca and co-authors (paper not yet available ) test the effectiveness of various strategies to decide what Boston restaurants should be targeted with health inspections. They show that recommendations from a complex machine learning algorithm outperforms the rankings generated by human inspectors. Interestingly, they also detect high levels of inspector non-compliance with AI recommendations, suggesting that workers don't trust these systems
- Adair Morse and co-authors analyze the impact of "fintech" AI systems in mortgage lending discrimination, finding that these systems tend to reduce - although not eliminate - discrimination against Latinx and African-American borrowers compared with face-to-face lenders, both in terms of the interest rates charged and the loan approval rates. However, AI systems still discriminate by identifying proxies for protected characteristics in the data. This shows how the adoption of AI can help tackle old problems (human prejudice) while introducing new ones (algorithmic bias).
Using AI to research AI
AI techniques have much to contribute to economics studies that often seek to detect causal patterns in data. Susan Athey surveyed these opportunities in the inaugural Economics of AI conference, with a particular focus on how machine learning can be used to enhance existing econometric methods.
Several papers mentioned above explored new data sources and methods along these lines, for example using big datasets from LinkedIn and Uber, and online experiments to test how UberX drivers react to informational nudges. At Nesta, we are analyzing open datasets with machine learning methods to map AI research.
Although these methods open up new analytical opportunities, they also raise challenges around reproducibility, particularly when the research relies on proprietary datasets that cannot be shared with other researchers (with the added risk of publication bias if data owners are able to control what findings are released), and ethics, for example around consent for participation in online experiments. Some of these challenges can be addressed by sharing the data and code used during the analysis, and developing ethical guidelines for the application of new methods.
Future avenues for the Economics of AI
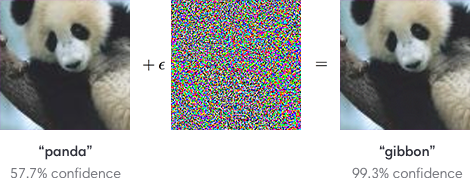
Having summarized key themes and papers from the conference, I focus on some questions that I felt were missing from the discussion.
Modeling AI failure
Macro studies of the impact of AI assume AI will increase productivity as long as businesses undertake the necessary complementary investments. They pay little attention to new issues created by AI such as algorithmic manipulation, bias and error, worker non-compliance with AI recommendations, or information asymmetries in AI markets. These factors could reduce AI's impact on productivity (making it mediocre and therefore predominantly labor displacing), increase the need to invest in new complements such as AI supervision and moderation, hinder trade in potentially dodgy AI products and services, and have important distributional implications, for example through algorithmic discrimination of vulnerable groups.
Macro research on AI should start to consider explicitly these complex aspects of AI adoption and impact, rather than hiding them in the black box of AI-complementing intangible investments and/or assuming that they are somehow exogenous to AI deployment. As an example, in previous work, I started to sketch what such a model could look like if we take into account the risk of algorithmic error in different industries, and the investments in human supervision required to manage it.
Modeling AI progress
In general, the research presented at the Economics of AI conference modeled AI as an external shock to the economy, in some cases explicitly as with Daniel Rock’s study of the impact of TensorFlow's release on firms' market value. However, AI progress is, itself, an economic process whose analysis should be part of the Economics of AI agenda.
In his conference dinner speech, Jack Clark from OpenAI described key trends in AI R&D: we are witnessing an "industrialization of AI" as corporate labs, big datasets and large scale IT infrastructures become more important in AI research, and at the same time a "democratisation of AI" as open-source software, open data and cloud computing make it easier to deploy state of the art AI systems. These changes have important economic implications. For example, the fact that researchers in academia increasingly need to collaborate with the private sector to access the data and compute required to train state of the art AI systems could skew this research or reduce its public value. Meanwhile, the diffusion of AI research through open channels creates important challenges for regulators who need to monitor compliance in an environment where adopting dangerous AI technologies is as simple as downloading and installing some software from GitHub. Few if any of the papers presented at the conference addressed these questions.
Future work could fill these gaps by developing formal models of AI progress through an AI production function that uses data, software, computational infrastructure and skilled labor to produce AI systems. In this paper, Miles Brundage started outlining qualitatively what that model could look like. This model could be operationalized using data from open and web sources and initiatives to measure AI progress from the Electronic Frontier Foundation (EFF) and the Papers with Code project in order to study the structure, composition and productivity of the AI industry, and how it supplies AI technologies and knowledge to other sectors. Recent work by Felten, Raj and Seamans where they use the EFF indicators to link advances in AI technologies with jobs at risk of automation illustrates how this kind of analysis could help forecast the economic impacts of AI progress and inform policy.
Studying the direction of AI inventive activity
Maintaining diversity in the range of technologies that are explored can be beneficial, specially when we do not know what their pros and cons are. However, as Daron Acemoglu argued in this 2011 paper, the market will under-supply alternatives to a dominant technology if researchers are not able to capture the benefits of sustaining technological diversity.
Most of the research presented at the NBER conference adopted a "monolithic" definition of AI equating it with the deep learning paradigm that dominates the field today, and neglecting concerns about the limitations of this approach. Yet as Gary Marcus has argued in recent work, other techniques may be necessary to make AI systems more robust and suitable for high-stakes domains like health.
Could lack of technological diversity become a problem in the AI field? Lack of diversity in the AI research workforce, and the increasing influence of the private sector in setting AI research (and ethical) agendas as part of the industrialization of AI research suggest that this could be a problem, but the evidence base is lacking. We need more research to measure AI's technological diversity and how it is shaped by the goals, preferences and agendas of the people and organizations involved in it. This is an active area of research for my team in Nesta. We just published an analysis of the thematic composition of AI research providing the foundation for an analysis of the evolution of diversity and its drivers in future work.
Remembering the political economy of AI
In the inaugural Economics of AI conference, Tratjenberg and Korinek and Stiglitzasked who will benefit and who will suffer when AI arrives, whether AI deployment could become politically unacceptable, and what policies should be put in place to reduce the societal costs of AI. More recently, Daron Acemoglu and Pascual Restrepo expressed concerns that the AI industry might be building "the wrong kind of AI" because it does not take into account the indirect impact of AI (for example in terms of labor market disruption) and because some of its leaders are biased in favor of mass automation regardless of its downsides. These important questions were largely absent from the debate in Toronto, yet economists need to formalize and operationalize models of the distributional impacts of AI and its externalities in order to inform policies to ensure that its economic benefits are widely shared and reduce the risk of a public backlash against it.
Conclusion: Think Internet, not Skynet
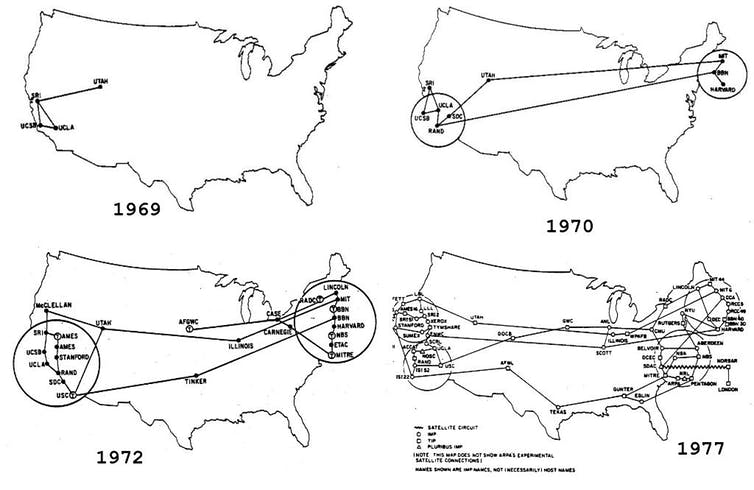
For me, the biggest takeaway from last year's Economics of AI conference was that AI impacts will be more complex and take longer to appear than what some newspaper headlines might lead us to expect. Jobs will evolve and adapt in response to AI systems rather than disappearing completely. Firms will experiment to discover how to create value from AI. Some of these experiments will fail, or prove that the adoption of AI is uneconomical. Some firms will learn from these failures and others will try again. Skills shortages, tighter regulation and consumer fears will slow down the adoption of some AI systems and favour others. The adoption of AI in an industry or firm will create sub-industries intent on manipulating it and lead to unexpected outcomes, and new changes in response.
In other words, the future of AI in the economy will resemble the Internet more than Skynet: it will be complicated. Prediction machines not only increase the amount of decisions we are able to make based on AI recommendations, but also the amount of decisions that we need to make, as participants in the economy and as a society, about what AI technologies to develop, where to adopt them and how, and how to manage their impacts. As the timely discussions in the latest Economics of AI conference showed, some of the best economists in the world are working hard to generate theories and evidence to inform these decisions.
Author Bio
Juan Mateos-Garcia is Director of Innovation Mapping at Nesta, the UK innovation foundation. There, he leads a team using novel data sources and methods to measure and map innovation in various industries and technologies including AI. Juan is an Economist with a MSc in Science and Technology Policy from the University of Sussex. Find him on Twitter
Acknowledgments
Special thanks to the organizers of the Economics of AI conference for creating a stimulating forum to discuss economics research on AI and its policy implications. Thanks to Mirantha Jayathilaka for editing this piece
Cover image source.
Citation
For attribution in academic contexts or books, please cite this work as
Juan Mateos-Garcia, "A Speech-To-Text Practitioner’s Criticisms of Industry and Academia", The Gradient, 2020.
BibTeX citation:
@article{mateosgarcia2020aieconomics,
author = {Mateos-Garcia, Juan},
title = {The Economics of AI Today},
journal = {The Gradient},
year = {2020},
howpublished = {\url{https://thegradient.pub/the-economics-of-ai-today/ } },
}
If you enjoyed this piece and want to hear more, subscribe to the Gradient and follow us on Twitter.