In a landmark paper [1], Alan Turing proposed a test to evaluate the intelligence of a computer. This test, later aptly named the Turing Test, describes a person interacting with either a computer or another human through written notes. If the person cannot tell who is who, the computer passes the test and we can conclude it displays human-level intelligent behavior.
In another landmark paper [2] a bit over a decade later, Gwilym Lodwick, a musculoskeletal radiologist far ahead of his time, proposed using computers to examine medical images and imagined
"[...] a library of diagnostic programs effectively employed may permit any competent diagnostic radiologist to become the equivalent of an international expert [...]."
He coined the term computer-aided diagnosis (CAD) to refer to computer systems that help radiologists interpret and quantify abnormalities from medical images. This led to a whole new field of research combining computer science with radiology.
With the advent of efficient deep learning algorithms, people have become more ambitious and the field of computer "aided" diagnosis is slowly changing to "computerized" diagnosis. Geoffrey Hinton even went as far as stating that we should stop training radiologists immediately because deep neural networks will likely outperform humans in all areas, five or ten years from now.
For a computer to be accepted as a viable substitute for a trained medical professional, however, it seems fair to require it to surpass or at least perform on par with humans. This "Turing test for medical image analysis", as it is often referred to, is close to being solved for some medical problems but many are still pending. What explains this difference? What makes one problem harder than the other?
This article presents an informal analysis of the learnability of medical imaging problems and decomposes it into three main components:
- The amount of available data
- The data complexity
- The label complexity
We will subsequently analyze a set of papers, all claiming human-level performance on some medical image analysis tasks to see what they have in common. After that, we will summarize the insights and present an outlook.
Learning from medical data
Machine learning algorithms essentially extract and compress information from a set of training examples to solve a certain task. In the case of supervised learning, this information can come from different sources: the labels and the samples. Roughly, the more data there is, the easier the problem is to learn. The less contaminated the data is, the easier the problem is to learn. The cleaner the labels are, the easier the problem is to learn. This is elaborated on below.
1. Amount of available data
Training data is one of the most important elements for a successful machine learning application. For many computer vision problems, images can be mined from social media and annotated by non-experts. For medical data, this is not the case. It is often harder to obtain and requires expert knowledge to generate labels. Therefore, large publicly-available and well-curated datasets are still scarce.
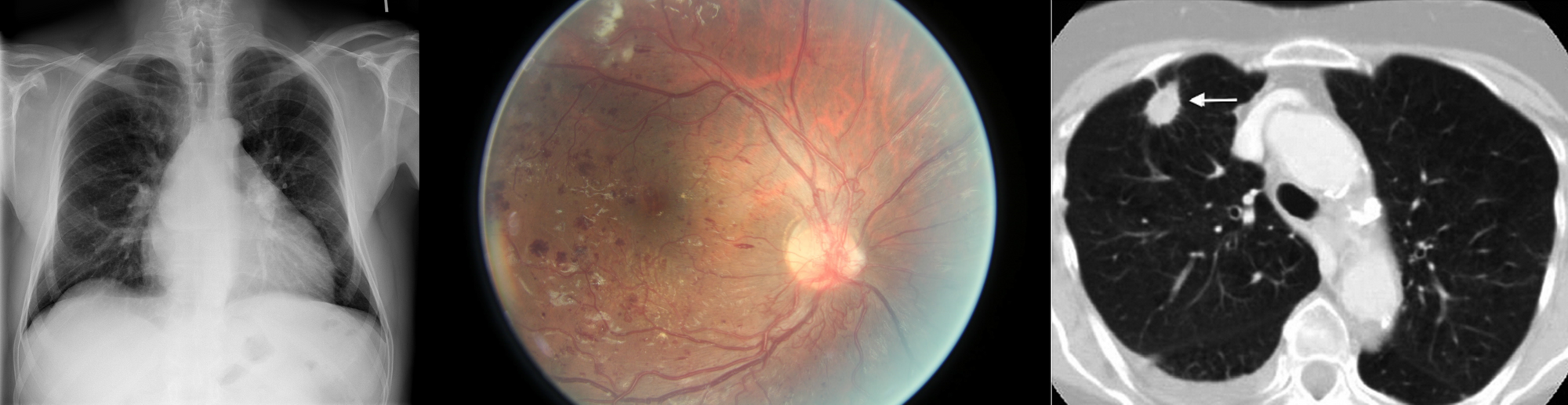
In spite of this, several (semi) publicly available datasets have emerged, such as the Kaggle diabetic retinopathy challenge [3], the LIDC-IDRI chest CT dataset [4], the NIH chest X-ray dataset [5], Stanford’s chest X-ray dataset [6], Radboud’s digital pathology datasets [7], the HAM10000 skin lesion dataset [8] and the OPTIMAM mammography dataset [9]. Example images of these datasets are shown in Figure 1. The availability of these public datasets has accelerated the research and development of deep learning solutions for medical image analysis.
2. Data complexity
Although the amount of available data is very important and often a limiting factor in many machine learning applications, it is not the only variable. Irrespective of the size of the data, feeding random noise to a statistical model will not result in the model learning to differentiate cats from dogs. We separate the data complexity into three types: image quality, dimensionality, and variability.
2.1. Image quality
Image quality has been an important topic of research from the beginning of medical image analysis and there are several aspects that define it [11]. The signal to noise ratio is often used to characterize quality, though other aspects such as the presence of artifacts, motion blur, and proper handling of the equipment also determine quality [12]. For example, in the case of mammography, the breast can be misplaced in the machine resulting in poor visibility of important structures. The image can be under or overexposed and artifacts such as pacemakers, implants or chemo ports can make the images hard to read. Examples of these are shown in Figure 2.
There are many definitions of signal to noise ratio. From an ML perspective, one of the easiest is the fraction of task-relevant information over the total information in the image.
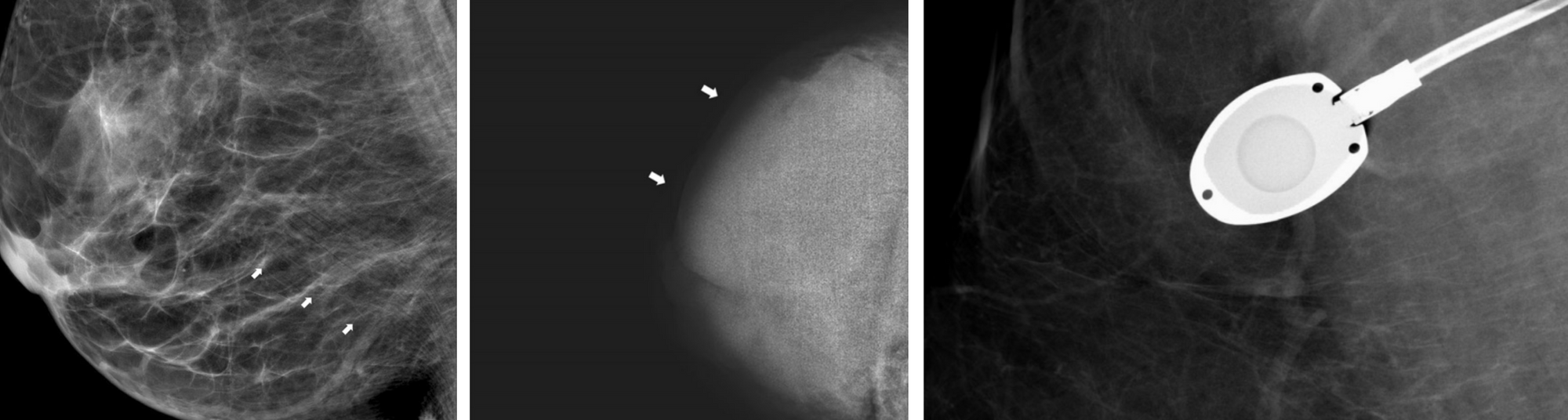
2.2 Dimensionality
The curse of dimensionality, a well-known problem from machine learning theory, describes the observation that with every feature dimension added, exponentially more data points are needed to get a reasonable filling of the space. Part of the curse has been lifted by the introduction of weight sharing in the form of convolutions and using very deep neural networks. However, medical images often come in far higher dimensional form than natural images (e.g., ImageNet has images of only 224x224).
2D images with complex structures, 3D images from MRI and CT, and even 4D images (for instance, a volume evolving over time) are the norm. Complex data structures typically mean you have to handcraft an architecture that works well for the problem and exploit structures that you know are in the data to make models work, see for example [13, 14].
2.3 Data variability
Scanners from different manufacturers use different materials, acquisition parameters, and post-processing algorithms [15, 16]. Different pathology labs use different stains [17]. Sometimes even data from the same lab taken with the same scanner will look different because of the person who handled the acquisition. These add complexity to the learning problem, because the model needs to become invariant, or engineers have to compensate for it by handcrafting preprocessing algorithms that normalize the data, employ heavy data augmentation or change network architectures to make them invariant to each irrelevant source.
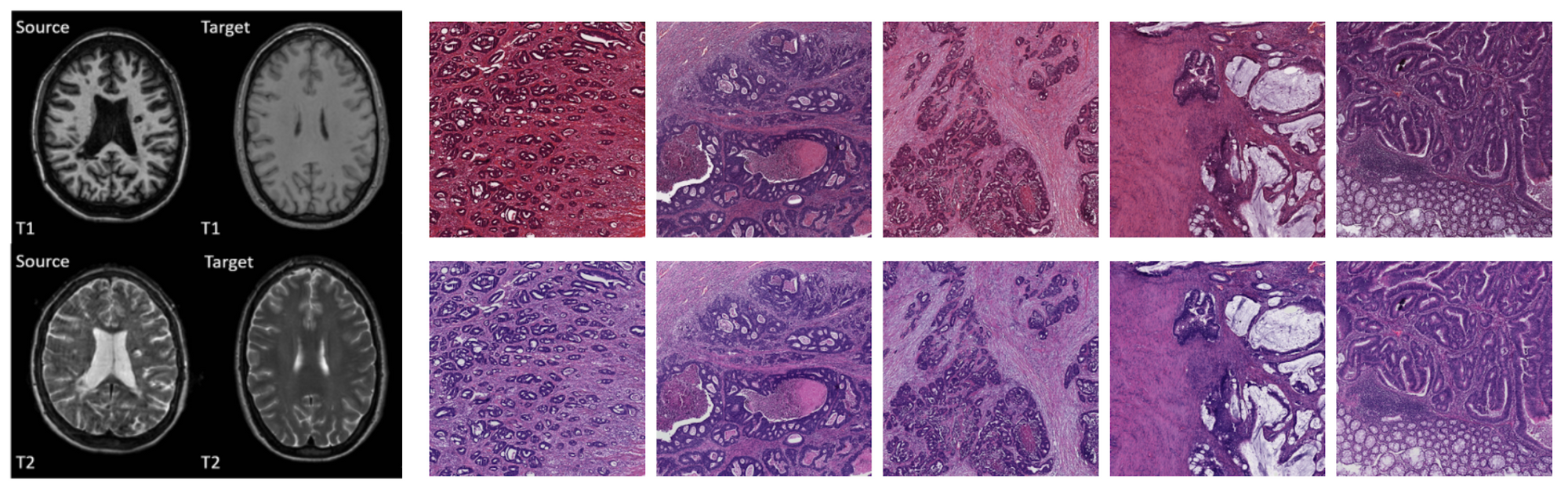
3. Label complexity
Although unsupervised methods are rising in popularity, most problems are still tackled using supervision, in which case we have another source of complexity: the labels. We again separate three factors: (1) the label granularity which describes the detail at which the labels are provided, (2) the class complexity which refers to the complexity of the output space, and (3) the annotation quality, which relates to the label noise.
3.1 Label granularity
Data can be annotated at different levels of granularity. In general, the granularity of the label used during training, does not have to be the same as the granularity generated during inference. For instance, segmentation maps (a label for every pixel in the image) can be generated when training on image-level labels only (e.g., multiple-instance learning), reports can be generated when training on class labels only, class-level labels can be generated when training on pixel-level labels, etc. An overview is given in Figure 4 and the configurations are elaborated on below.
- Pixel level labels - Segmentation: In this setting the algorithm is supplied with contour annotations and/or output labels for every pixel in the case. In earlier medical imaging systems, segmentations were often employed as a form of preprocessing for detection algorithms, but this became less common after the ImageNet revolution in 2012. Segmentation algorithms are also used for quantification of abnormalities which can again be used to get consistent readings in clinical trials or as preparation for treatment such as radiotherapy. For natural images, a distinction is often made between instance-based and semantic segmentation. In the first case, the algorithm should provide a different label for every instance of a class and in the second case, only for each class separately. This distinction is less common in biomedical imaging, where there is often only one instance of a class in an image (such as a tumor), and/or detecting one instance is enough to classify the image as positive.
- Bounding boxes - Detection: Instead of providing/generating a label for every pixel in the image, the annotator can also just draw a box around a region of interest. This can save time during annotation, but may provide lesser information: a box has four degrees of freedom, a contour usually more. It is difficult to tell the effect this has on algorithms.
- Study level labels - Classification: Yet another simpler way to annotate would be to just flag cases with a specific abnormality. This makes a lot of sense for problems where the whole study is already a crop around the pathology in question, such as skin lesion classification and classifying abnormalities in color fundus imaging. This is much harder for other problems, such as the detection of breast cancer where a study is typically a time series of four large images and pathologies only constitute a fraction of the total study size. The algorithm first has to learn which parts of the image are relevant and then learn the actual features.
- Reports - Report generation: Again, a simpler way to annotate is to use labels extracted directly from the radiology reports, instead of annotators. This type of label is sometimes referred to as silver standard annotation [18]. Learning from this data is likely difficult because natural language processing needs to be employed first, to extract labels from the report, potentially increasing label noise. Within this type of label, we can make one more distinction: structured or unstructured reports. In the first case, the doctor was constrained to a specific standard, in the latter case the doctor was free to write what they wanted. Whichever is best is an interesting debate: unstructured reports have the potential to contain more information but are more difficult to parse.
3.2 Class complexity
- Number of disease classes
There is a difference between classifying images into two different classes or 1000 different classes. The first case means splitting the space in two separable regions, the latter into 1000, which is likely harder.
- Co-occurring symptoms
In many applications, for example, detecting abnormalities in chest X-ray, one sample can have multiple labels at the same time. This is illustrated in Figure 6, which shows a circular diagram of the co-occurrence of classes in the Chest X-ray 8 dataset.
3.3 Label noise - annotation quality
Apart from the granularity of annotation, there is one more factor that can influence learnability and the eventual performance of the AI algorithm: the accuracy of the annotation. There are essentially two ways to handle annotations for most medical imaging problems: using a human reference or some other test that is known to be more accurate than the human.
- Reader based labels - Label limited problems
If the labels are generated by medical professionals there is often a significant amount of inter-reader variability (different readers give different labels for the same sample) and intra-reader variability (the same reader gives different labels for the same sample, when annotated at different times). This disagreement results in label noise which makes it more difficult to train good models.
An important corollary of this is that using these labels to evaluate the model, it will be hard to show you actually outperform humans. Problems using this type of label are therefore label-limited: even if we have the best possible architecture, an infinite amount of training data, and compute power, we will never be able to outperform humans by a large margin, since they define the ground truth.
- Test-based labels - Data limited problems
Imaging is often used as a first test because it is typically cheap and non-invasive. If something suspicious is found, the patient is subjected to more tests that have a higher accuracy. For example, in chest X-ray images, we sometimes have microbial culture to confirm the patient has tuberculosis. For the detection of tumors, biopsies and histopathological outcomes can be employed. These types of labels provide a less noisy and more accurate ground truth.
Problems using these labels are data-limited: if we just give an algorithm enough data, train it well and use the right architecture it should be able to outperform humans at some point, provided the second test is better than the human reading the image.
Analysis of papers
To get a better understanding of the state-of-the-art and what more is in store, we will analyze a few recent papers. This is by no means intended as an exhaustive list nor do we claim the results in the papers are accurate. All claims about (super) human performance become more trustworthy in prospective clinical trials, when data and source code are made publicly available, or when methods are evaluated in challenges.
The table below presents a summary of each paper, the data the model was trained on, and the labels that were used for the problem. For a more rigorous, clinically oriented overview, please refer to [19] and [20].
Modality | Paper | Summary | Data | Labels |
---|---|---|---|---|
Chest X-ray | Rajpurkar, Pranav, et al. "Chexnet: Radiologist-level pneumonia detection on chest x-rays with deep learning." arXiv preprint arXiv:1711.05225 (2017). | A model for the automatic detection of (radiological evidence of) pneumonia. Human performance is claimed by comparing it to the radiological label of several radiologists | Around 120K frontal view X-rays. 2D single view images downscaled to 224 x 224. | The dataset contains labels for 14 pathologies, which are extracted using NLP, therefore, silver standard labels were used. However, only signs of pneumonia or not is used as a label. The problem is label limited, the system is compared against labels defined by radiologists. |
Chest X-ray | Putha, Preetham, et al. "Can Artificial Intelligence Reliably Report Chest X-Rays?: Radiologist Validation of an Algorithm trained on 2.3 Million X-Rays." arXiv preprint arXiv:1807.07455 (2018). | Nine different findings were detected using different models trained for each finding. Human performance was shown for about four pathologies. | Around 120K frontal view X-rays. 2D single view images downscaled to 224 x 224. A total of 1.2 million chest X-rays were used, downscaled to an unspecified size. Normalization techniques were applied to reduce variation. | The labels were extracted using NLP from the radiology reports and are therefore silver standard labels. Multi-class labels, treated as a binary classification problem. |
Chest X-ray | Irvin, Jeremy, et al. "Chexpert: A large chest radiograph dataset with uncertainty labels and expert comparison." Proceedings of the AAAI Conference on Artificial Intelligence. Vol. 33. 2019. | A model was trained to discriminate between 14 different findings. Human performance is shown for five of those abnormalities. | About 220K scans, 2D images, single view, downscaled to 320 x 320 | Labels extracted using NLP (silver standard labels), label limited. One model is trained for each pathology In addition, uncertainty based labels were used. |
Chest X-ray | Hwang, Eui Jin, et al. "Development and validation of a deep learning–based automated detection algorithm for major thoracic diseases on chest radiographs." JAMA network open 2.3 (2019): e191095-e191095. | An algorithm is proposed to detect four abnormalities in chest radiographs. The model is compared to 15 physicians and shown to have higher performance | About 55K radiographs with normal findings and 35K with abnormal findings where used to develop the model. | Binary classification, four abnormalities merged into one. Mix of histopathology/external test and radiological labels were used. Largely a label limited problem. |
Chest X-ray | Nam, Ju Gang, et al. "Development and validation of deep learning–based automatic detection algorithm for malignant pulmonary nodules on chest radiographs." Radiology 290.1 (2019): 218-228. | A model was trained to detect malignant pulmonary nodules on chest radiographs. The model was compared to 18 human readers and showed superior performance to the majority of them. | 42K radiographs, 8000 with nodules in total were used for development | Both pixel level and image level labels were used for training. The malignancies were biopsy proven, this is therefore a daa limited problem. The problem was phrased as a binary classification. |
Chest CT | Ardila, Diego, et al. "End-to-end lung cancer screening with three-dimensional deep learning on low-dose chest computed tomography." Nature medicine 25.6 (2019): 954-961. | A deep neural network is shown to outperform 6 human readers in classifying lung cancer in low dose CT | 42K low dose CT scans, cropped and resized to 240 x 240 x 240. (3D data) | Different components of the model were trained on either the region level or case level labels (outcome determined by biopsy).Biopsy proven malignancies were used (data limited). Binary classification |
Mammography | McKinney, Scott Mayer, et al. "International evaluation of an AI system for breast cancer screening." Nature 577.7788 (2020): 89-94. | A DNN is trained to detect breast cancer in screening mammography. The model is compared to a UK and US dataset and shown to outperform radiologists on both sets. | The model was trained on about 130K mammograms. A mammogram comprises four views, two of each breast. | Binary classification. The ground truth was determined based on histopathology reports. This is a data limited problem. |
Dermatology | Esteva, A., Kuprel, B., Novoa, R.A., Ko, J., Swetter, S.M., Blau, H.M. and Thrun, S., 2017. Dermatologist-level classification of skin cancer with deep neural networks. Nature, 542(7639), pp.115-118. | A model is proposed that classifies dermatology images into malignant or benign and is compared to 21 certified dermatologists. | The model was developed on roughly 130K images. The dataset consisted of both color images from a regular camera and dermoscopy images. Images were scaled to 299 x 299. | The model was trained on a combination of biopsy proven and radiologists labeled cases. It is therefore a label limited problem. The model was trained using many different classes, comparison to humans done on a binary classification problem |
Dermatology | Han, S.S., Kim, M.S., Lim, W., Park, G.H., Park, I. and Chang, S.E., 2018. Classification of the clinical images for benign and malignant cutaneous tumors using a deep learning algorithm. Journal of Investigative Dermatology, 138(7), pp.1529-1538. | A model is proposed that can classify 12 different cutaneous tumors. The performance of the algorithm was compared to a group of 16 dermatologists, using biopsy proven cases only. The model performed comparable to the panel of dermatologists. | The network was developed on about 17K images. The set comprised color images from a regular camera of varying size (size used in CNN not specified) | Most pathologies were biopsy confirmed this is therefore a data limited problem. The final model used 12 class classification. |
Pathology | Bejnordi, B.E., Veta, M., Van Diest, P.J., Van Ginneken, B., Karssemeijer, N., Litjens, G., Van Der Laak, J.A., Hermsen, M., Manson, Q.F., Balkenhol, M. and Geessink, O., 2017. Diagnostic assessment of deep learning algorithms for detection of lymph node metastases in women with breast cancer. Jama, 318(22), pp.2199-2210. | A challenge was held to detect breast cancer metastasis in lymph nodes (CAMELYON16). The submitted algorithms were compared to a panel of 11 pathologists that read the slides under normal conditions (time constrained) and unconstrained. The top 5 algorithms all outperformed the readers under time constrained conditions and performed comparable in a non-time time constrained setting. | A total of 270 digital pathology slides were used for development (110 with and 160 without node metastasis) | It was phrased as a binary classifications. Labels were generated by pathologists and this is therefore a label limited problem. |
Pathology | Bulten, Wouter, et al. "Automated deep-learning system for Gleason grading of prostate cancer using biopsies: a diagnostic study." The Lancet Oncology (2020). | A model was trained to perform Gleason grading of prostate cancer in digital pathology slides. The algorithm was compared to 115 pathologists and shown to outperform 10 of them. | Around 5000 digital pathology slides (color images). | Five class classification: labels were provided by experts/reports and therefore this is a label limited problem. The model was trained using semi-automatic labelling, where labels were extracted from reports and corrected if they deviated too much from an initial models' prediction. |
Color fundus | Gulshan, Varun, et al. "Development and validation of a deep learning algorithm for detection of diabetic retinopathy in retinal fundus photographs." Jama 316.22 (2016): 2402-2410 | A CNN was trained to detect diabetic retinopathy and diabetic macular edema. The algorithm was compared to 7 - 8 opthamologists respectively and was found to perform on par. | About 130K color fundus scans. Resolution used for the model was not mentioned | Binary labels: referable diabetic retinopathy or diabetic macular edema. Labels are provided by the expert (label limited problem). |
Optical coherence tomography | De Fauw, Jeffrey, et al. "Clinically applicable deep learning for diagnosis and referral in retinal disease." Nature medicine 24.9 (2018): 1342-1350 | A method is proposed to classify retinal diseases in OCT images. The model is compared to four retina specialists. It matched two and outperformed to others | Around 15K OCT scans, 3D data of 448 x 512 x 9 | Combination of segmentation and classification. The segmentation was trained on 15 classes.The output of a segmentation algorithm is used as input for a network that outputs one of four referral diagnoses. Labels are provided by expert (label limited problem). |
Discussion
Successes
The number of successful AI applications has drastically increased in the past couple of years. More specifically, high-throughput applications that carry a relatively low risk for the patient, such as detection of various diseases in chest X-ray, diabetic retinopathy detection in color fundus scan and detection of breast cancer in mammography seem to be popular. Note that in most of the applications, especially in chest X-ray analysis, AI algorithms have been successful only for a limited number of pathologies and will likely not replace the human completely any time soon.
More concretely, things that go well are:
- Small images such as color fundus scans or dermatology images. Making ImageNet models work well on these problems is straightforward, there are no memory issues due to the relatively lower dimensionality of the data and changes in architecture are typically not necessary. In most cases, one sample is already a crop around the pathology to be classified, so simple classification architectures can be employed.
- Diseases in modalities with lots of available data (because for instance the diseases detected using the images are common, the images are used to help diagnose multiple diseases, the images are cheap or little to non-invasive) such as diabetic retinopathy from color fundus, TB in chest X-ray and breast cancer in mammograms. More data simply means better performance, it is not surprising that domains where a lot of data is available are solved first.
- Binary classification/detection problems such as the detection of single diseases in chest X-ray or cancer in mammograms.
Challenges
In spite of this success, there are many challenges left. The biggest are:
- Applications such as MRI where there is a lot of noise, large variability between different scanners/sequences and a lack of data (an MRI scan is costly and the diseases used to detect it are less common). This is compounded by the fact that due to the volumetric nature of the data, it may be harder to annotate and developers therefore rely on study level labels or methods that can handle sparse annotations.
- Detecting multiple diseases, especially rare disease. Even though there are big datasets for X-ray, it seems many algorithms still struggle to accurately distinguish all abnormalities in chest X-ray images at human level. This may be because some categories are underrepresented or because of the additional label noise introduced by using silver standard labels.
- Complex output such as segmentation or radiology reports. Except for a few applications that use it as an intermediate step, we found no papers that show clear (super)human segmentation or report generation. Also note that both of these problems are hard to evaluate because of the nature of the labels.
- Problems with complex input such as 3D/4D data, perfusion, contrast enhanced data.
- Applications in highly specialized modalities that are not commonly used [25], such as PET and SPECT scans (medical scans that make use of radioactive substances to measure tissue function).
There is still a long way to go for AI to completely replace radiologists and other medical professionals. Below are a few suggestions.
1. Getting more data
This is a brute-force approach, but often the best way to get machine learning systems to work well. We should incentivize university hospitals sitting on data gold mines to make their data public and share it with AI researchers. This may sound like an unrealistic utopia, but there may be a business model where this can work. For example, patients could own their data and receive money for sharing an anonymized extract with a private institute.
In the long run, collecting massive amounts of data for every disease will not scale though, so we also have to be smart with the data we do have.
2. Using data more efficiently
- Standardize data collection: Anyone who worked on actually applying AI to real world problems, knows most of the sweat and tears still goes into the collection of data, cleaning it and standardizing it. This process can be made much more efficient. Hospital software systems often make sure the data format is not compatible with competitors to "lock you in". This is similar to how Apple and Microsoft try to prevent you from using other software. Although this may make sense from a business perspective, this is hindering health care. In the ideal world, every hospital should use the same system for collecting data or conversion should be trivial. Algorithm vendors should provide the raw data and/or conversion functions between different vendors so that standardization between vendors becomes easier and generalization of algorithms is less of a problem.
- Methods working with less data - few shot learning, meta learning: Long tail samples are a well known problem in real world machine learning applications such as autonomous driving and medical diagnosis. A similar problem exists for modalities and pathologies: the distribution of the number of recorded images per modality also follows a Pareto distribution. Over a billion chest X-rays are recorded annually, approx 100 - 200 million mammograms, but only a couple of million specialized scans such as PET and SPECT. This is illustrated in Figure 7. Collecting large numbers of examples for every individual problem clearly does not scale. Methods such as few shot learning and meta learning [21 - 24] that aim to use data efficiently and learn across tasks, instead of just a single task will likely be crucial in tackling the full spectrum of the long tail of modalities.
3. Methods working with less labels - semi-supervised and active learning
Using experts to perform annotations for all diseases and all modalities also does not scale. Medical data can not simply be annotated by verifying a doctor is not a robot, like Google insists on doing for their search engines. Methods dealing efficiently with sparse annotations such as semi-supervised and active learning will have to be employed. The successful application of silver standard labels in chest X-ray and digital pathology are impressive and could further be explored.
Conclusion
Hinton’s claim that a radiologist’s work will be replaced in the next few years seems far off, but the progress of AI is nevertheless promising. Digital Diagnostics [26] is already deploying a fully autonomous AI system for grading diabetic retinopathy in color fundus images. Several applications such as the detection of skin lesions, breast cancer in mammography and various symptoms of diseases in chest X-ray are becoming more mature and may soon follow suit.
A complete transition from Lodwick’s dream of a computer "aided" diagnosis to the more ambitious "computerized" diagnosis for all diseases in all modalities will likely still be a few decades away. If there is one thing the COVID-19 pandemic taught us, however, is that exponential growth is often underestimated. Computational power, available data and the number of people working on medical AI problems keeps increasing every year (especially in data rich countries such as China and India), speeding up development. On the other hand, progress in machine learning applications is logarithmic: it is easy to go from an AUC of 0.5 to 0.7, but completely covering the long tail, i.e., going from an AUC of 0.95 to 0.99 takes the most effort. The same will likely hold true for the long tail in modalities.
Turing postulated the requirement for solving the imitation game will be mainly about programming. For medical imaging problems, it will be mainly about data. Most image reading problems are simple image in - label out settings that can already be solved using current technology. Beyond that horizon, many new challenges will arise as a radiologists job is about much more than just reading images. Completely replacing radiologists is close to solving artificial general intelligence. Combining imaging modalities, images with lab work, anamnesis and genomic profiles of patients will likely keep us busy for decades to come.
References
[1] Turing, A.M., 2009. Computing machinery and intelligence. In Parsing the Turing Test (pp. 23-65). Springer, Dordrecht.
[2] Lodwick, G.S., 1966. Computer-aided diagnosis in radiology: A research plan. Investigative Radiology, 1(1), pp.72-80.
[3] https://www.kaggle.com/c/diabetic-retinopathy-detection
[4] Armato III, S.G., McLennan, G., Bidaut, L., McNitt‐Gray, M.F., Meyer, C.R., Reeves, A.P., Zhao, B., Aberle, D.R., Henschke, C.I., Hoffman, E.A. and Kazerooni, E.A., 2011. The lung image database consortium (LIDC) and image database resource initiative (IDRI): a completed reference database of lung nodules on CT scans. Medical physics, 38(2), pp.915-931.
[5] Wang, X., Peng, Y., Lu, L., Lu, Z., Bagheri, M. and Summers, R.M., 2017. Chestx-ray8: Hospital-scale chest x-ray database and benchmarks on weakly-supervised classification and localization of common thorax diseases. In Proceedings of the IEEE conference on computer vision and pattern recognition(pp. 2097-2106).
[6] Irvin, J., Rajpurkar, P., Ko, M., Yu, Y., Ciurea-Ilcus, S., Chute, C., Marklund, H., Haghgoo, B., Ball, R., Shpanskaya, K. and Seekins, J., 2019, January. Chexpert: A large chest radiograph dataset with uncertainty labels and expert comparison. In Thirty-Third AAAI Conference on Artificial Intelligence.
[7] Bejnordi, B.E., Veta, M., Van Diest, P.J., Van Ginneken, B., Karssemeijer, N., Litjens, G., Van Der Laak, J.A., Hermsen, M., Manson, Q.F., Balkenhol, M. and Geessink, O., 2017. Diagnostic assessment of deep learning algorithms for detection of lymph node metastases in women with breast cancer. Jama, 318(22), pp.2199-2210.
[8] Tschandl, P., Rosendahl, C. and Kittler, H., 2018. The HAM10000 dataset, a large collection of multi-source dermatoscopic images of common pigmented skin lesions. Scientific data, 5, p.180161.
[9] Halling-Brown, M.D., Warren, L.M., Ward, D., Lewis, E., Mackenzie, A., Wallis, M.G., Wilkinson, L., Given-Wilson, R.M., McAvinchey, R. and Young, K.C., 2020. OPTIMAM Mammography Image Database: a large scale resource of mammography images and clinical data. arXiv preprint arXiv:2004.04742.
[11] Prince, J.L. and Links, J.M., 2006. Medical imaging signals and systems. Upper Saddle River, NJ: Pearson Prentice Hall.
[12] Choi, J.J., Kim, S.H., Kang, B.J., Choi, B.G., Song, B. and Jung, H., 2014. Mammographic artifacts on full-field digital mammography. Journal of digital imaging, 27(2), pp.231-236.
[13] Veeling, B.S., Linmans, J., Winkens, J., Cohen, T. and Welling, M., 2018, September. Rotation equivariant CNNs for digital pathology. In International Conference on Medical image computing and computer-assisted intervention (pp. 210-218). Springer, Cham.
[14] Pinckaers, H., van Ginneken, B. and Litjens, G., 2019. Streaming convolutional neural networks for end-to-end learning with multi-megapixel images. arXiv preprint arXiv:1911.04432.
[15] Mackin, D., Fave, X., Zhang, L., Fried, D., Yang, J., Taylor, B., Rodriguez-Rivera, E., Dodge, C., Jones, A.K. and Court, L., 2015. Measuring CT scanner variability of radiomics features. Investigative radiology, 50(11), p.757.
[16] He, Y., Carass, A., Zuo, L., Dewey, B.E. and Prince, J.L., 2020, October. Self domain adapted network. In International Conference on Medical Image Computing and Computer-Assisted Intervention (pp. 437-446). Springer, Cham.
[17] Ciompi, F., Geessink, O., Bejnordi, B.E., De Souza, G.S., Baidoshvili, A., Litjens, G., Van Ginneken, B., Nagtegaal, I. and Van Der Laak, J., 2017, April. The importance of stain normalization in colorectal tissue classification with convolutional networks. In 2017 IEEE 14th International Symposium on Biomedical Imaging (ISBI 2017) (pp. 160-163). IEEE.
[18] Agarwal, V., Podchiyska, T., Banda, J.M., Goel, V., Leung, T.I., Minty, E.P., Sweeney, T.E., Gyang, E. and Shah, N.H., 2016. Learning statistical models of phenotypes using noisy labeled training data. Journal of the American Medical Informatics Association, 23(6), pp.1166-1173.
[19] Nagendran, M., Chen, Y., Lovejoy, C.A., Gordon, A.C., Komorowski, M., Harvey, H., Topol, E.J., Ioannidis, J.P., Collins, G.S. and Maruthappu, M., 2020. Artificial intelligence versus clinicians: systematic review of design, reporting standards, and claims of deep learning studies. bmj, 368.
[20] Liu, X., Faes, L., Kale, A.U., Wagner, S.K., Fu, D.J., Bruynseels, A., Mahendiran, T., Moraes, G., Shamdas, M., Kern, C. and Ledsam, J.R., 2019. A comparison of deep learning performance against health-care professionals in detecting diseases from medical imaging: a systematic review and meta-analysis. The lancet digital health, 1(6), pp.e271-e297.
[21] Fei-Fei, L., Fergus, R. and Perona, P., 2006. One-shot learning of object categories. IEEE transactions on pattern analysis and machine intelligence, 28(4), pp.594-611.
[22] Ren, M., Triantafillou, E., Ravi, S., Snell, J., Swersky, K., Tenenbaum, J.B., Larochelle, H. and Zemel, R.S., 2018. Meta-learning for semi-supervised few-shot classification. arXiv preprint arXiv:1803.00676.
[23] Finn, C., Abbeel, P. and Levine, S., 2017. Model-agnostic meta-learning for fast adaptation of deep networks. arXiv preprint arXiv:1703.03400.
[24] Lake, B.M., Salakhutdinov, R. and Tenenbaum, J.B., 2019. The Omniglot challenge: a 3-year progress report. Current Opinion in Behavioral Sciences, 29, pp.97-104.
[25] https://www.england.nhs.uk/statistics/wp-content/uploads/sites/2/2018/03/Provisional-Monthly-Diagnostic-Imaging-Dataset-Statistics-2018-03-22.pdf[26] Abràmoff, M.D., Lavin, P.T., Birch, M., Shah, N. and Folk, J.C., 2018. Pivotal trial of an autonomous AI-based diagnostic system for detection of diabetic retinopathy in primary care offices. NPJ digital medicine, 1(1), pp.1-8.
Author Bio
Thijs Kooi is VP of radiology research at Lunit, a medical imaging AI startup in Seoul. Before joining Lunit, he was at Merantix and Vara, a breast imaging AI start-up in Berlin. He got a Ph.D. in computer aided diagnosis from Radboud University and a M.Sc. in artificial intelligence from the University of Amsterdam in The Netherlands. His research interest is machine learning applied to medical imaging and diagnosis.
Citation
For attribution in academic contexts or books, please cite this work as
Thijs Kooi, "Why skin lesions are peanuts and brain tumors a harder nut", The Gradient, 2020.
BibTeX citation:
@article{kooi2020medicalimages,
author = {Kooi, Thijs},
title = {Why skin lesions are peanuts and brain tumors harder nuts},
journal = {The Gradient},
year = {2020},
howpublished = {\url{https://thegradient.pub/why-skin-lesions-are-peanuts-and-brain-tumors-harder-nuts/} },
}
If you enjoyed this piece and want to hear more, subscribe to the Gradient and follow us on Twitter.